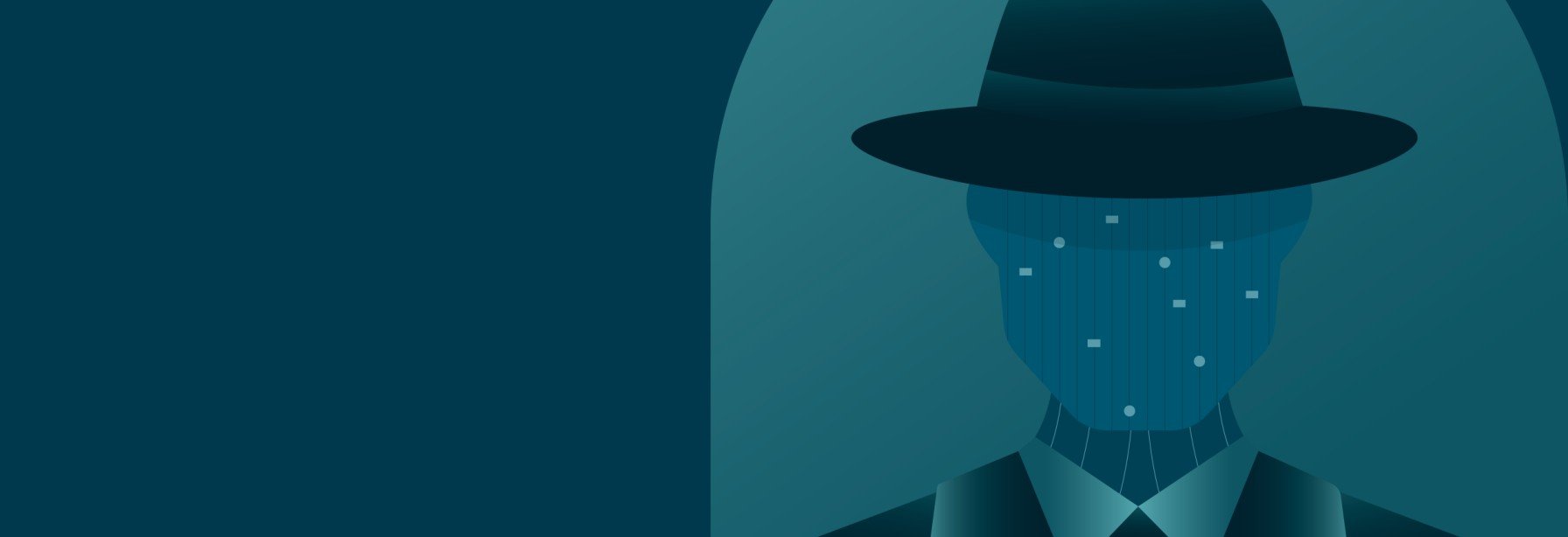
Artificial Intelligence and Retail Investing:
Scams and Effective Countermeasures
Executive Summary
Executive Summary
There has been a significant increase in the scale and breadth of artificial intelligence (AI) applications in retail investing. While these technologies hold promise for retail investors, they pose novel risks—in particular, the risk of AI increasing investor susceptibility to scams. The terms scams and frauds are often used interchangeably, however, for the purposes of this report, we define them as:
- Scams: Deceptive schemes intended to manipulate individuals to willingly provide information and/or money.
- Frauds: Deceptive schemes to gain unauthorized access to personal information and/or money without the targets’ knowledge or consent. Also defined as a broader, legal term that covers intentional dishonest activity, including scams.
The development and deployment of AI systems in capital markets raises important regulatory questions. The OSC is taking a holistic approach to evaluating the impact of AI systems on capital markets. This includes understanding how market participants are benefiting from the use of AI systems and understanding the risks associated with their use. It also includes analyzing how their use impacts market participants differently, whether investors, marketplaces, advisors, dealers, investment funds, and more. We hope our work in identifying scams and providing mitigation techniques will add to our growing body of publications relating to AI system deployment, which includes:
- Artificial Intelligence in Capital Markets – Exploring Use Cases in Ontario (October 10, 2023) [1]
- AI and Retail Investing (published on September 11, 2024)
This research was conducted by the OSC’s Research and Behavioural Insights Team with the assistance of the consultancy Behavioural Insights Team (BIT) Canada. Our research is structured into two components:
- A literature and environmental scan to understand current trends in AI-enabled online scams, and a review of system and individual-level mitigation strategies for retail investor protection.
- A behavioural science experiment to assess the effectiveness of two types of mitigation strategies in reducing susceptibility to AI-enhanced investment scams. This experiment also sought to assess whether AI technologies are increasing investor susceptibility to scams.
The literature and environmental scan revealed that malicious actors are exploiting AI capabilities to more effectively deceive investors, orchestrate fraudulent schemes, and manipulate markets, posing significant risks to investor protection and the integrity of capital markets. Generative AI technologies are “turbocharging” common investment scams by increasing their reach, efficiency, and effectiveness. New types of scams are also being developed that were impossible without AI (e.g., deepfakes and voice cloning) or that exploit the promise of AI through false claims of ‘AI-enhanced’ investment opportunities. Together, these enhanced and new types of scams are creating an investment landscape where scams are more pervasive and damaging, as well as harder to detect.
To combat these heightened risks, we explored proven and promising strategies to mitigate the harms associated with AI-enhanced or AI-related investment scams. We identified two sets of mitigations: system-level mitigations, which limit the risk of scams across all (or a large pool of) investors, and individual-level mitigations, which help empower or support individual investors in detecting and avoiding scams. At the individual level, we found promise in innovative mitigation strategies more commonly used to address political misinformation, such as “inoculation” interventions.
BIT Canada and the OSC’s Research and Behavioural Insights Team conducted an online, randomized controlled trial (RCT) to test the efficacy of promising mitigation strategies, as well as to better substantiate the harm associated with the use of generative AI by scammers. In this experiment, over 2000 Canadian participants invested a hypothetical $10,000 across six investment opportunities in a simulated, social media environment. Investment opportunities promoted ETFs, cryptocurrencies, as well as investment advising services (e.g., robo-advising or AI-backed trading algorithms), and included a combination of legitimate investment opportunities, conventional scams, and/or AI-enhanced scams. We then observed how participants allocated their funds across the investment opportunities. Some participants were exposed to one of two mitigation techniques, which were:
- Inoculation—a technique that provides high-level guidance on scam awareness prior exposure to the investment opportunities, and,
- A simulated web-browser plug-in that flagged potentially “high-risk” opportunities.
We found that:
- AI-enhanced scams pose significantly more risk to investors compared to conventional scams. Participants invested 22% more in AI-enhanced scams than in conventional scams. This finding suggests that using widely available generative AI tools to enhance fraudulent materials can make scams much more compelling.
- The “Inoculation” technique and web-browser plug-ins can significantly reduce the magnitude of harm posed by AI-enhanced scams. Both mitigation strategies we tested were effective at reducing susceptibility to AI-enabled scams, as measured through invested dollars. The “inoculation” strategy reduced investment in fraudulent opportunities by 10%, while the web-browser plug-in reduced investment by 31%.
Based on our findings from the experiment and the preceding literature and environmental scan, we conclude that:
- Widely available generative AI tools can easily enhance fraudulent materials for illegitimate investment opportunities—and that these AI enhancements can increase the appeal of these opportunities.
- System-level mitigations, followed by individual-level mitigations are both needed for retail investor protection against AI-related scams.
- Individual-level mitigations such as the “inoculation” technique and web-browser plug-ins can be effective tools at reducing the susceptibility of retail investors to AI-enhanced scams.
[1] https://oscinnovation.ca/resources/Report-20231010-artificial-intelligence-in-capital-markets.pdf
Introduction
Introduction
The rapid escalation in the scale and application of artificial intelligence (AI) has resulted in a critical challenge for retail investor protection against investment scams. To promote retail investor protection, we must understand how AI is enabling and generating investment scams, how investors are responding to these threats, and which mitigation strategies are effective. Consequently, we examined:
- The use of artificial intelligence to conduct financial scams and other fraudulent activities, including:
- How scammers use AI to increase the efficacy of their financial scams;
- How AI distorts information and promotes disinformation and/or misinformation;
- How effectively people distinguish accurate information from AI-generated disinformation and/or misinformation; and,
- How the promise of AI products and services are used to scam and defraud retail investors.
- The mitigation techniques that can be used to inhibit financial scams and other fraudulent activities that use AI at the system level and individual level.
Our report includes a mixed-methods research approach to explore each of these key areas:
- A literature and environmental scan to understand current trends in AI-enabled online scams, and a review of system and individual-level mitigation strategies to protect consumers. This included a review of 50 publications and “grey” literature (e.g., reports, white papers, proceedings, papers by government agencies, private companies, etc.) sources and 28 media sources. This scan yielded two prominent trends in AI-enabled scams: (1) Using generative AI to ‘turbocharge’ existing scams; and (2) Selling the promise of ‘AI-enhanced’ investment opportunities. This scan also summarized current system- and individual-level mitigation techniques.
- A behavioural science experiment to assess the effectiveness of two types of mitigation strategies in reducing susceptibility to AI-enhanced investment scams. This experiment also sought to quantify and confirm that AI technologies are increasing investor susceptibility to scams.
Desk Research
Desk Research
In this section, we discuss the emerging threats posed by AI use in online scams, and how to safeguard against them. The overarching goal is to better protect retail investors in the context of rapidly evolving, technology-enabled scams. We explore the current use of AI to conduct financial scams; how it is used by malicious actors to increase their volume, reach, and sophistication. We then describe a combination of both proven and promising strategies to mitigate the harms associated with AI-enabled or AI-related securities scams.
Our findings throughout this report are informed by academic publications, industry reports, media articles, discussions with subject matter experts, and an environmental scan of publicly reported financial scams. It is important to acknowledge that the application of AI to online scams is relatively new; accordingly, publicly available information is relatively scarce.
Advancements in AI are transforming the cybercrime landscape, making criminal behaviour easier to commit, more widespread, and more sophisticated than ever before. Nearly half of Canadians (49%) reported being targeted by some form of a fraud scheme in 2023.[2] This represents a 40% increase in digital fraud attempts originating in Canada compared to the same period in 2022.[3]
The Canadian Anti-Fraud Centre (CAFC) has reported a 40% increase in victim losses as a result of fraud and cybercrime, increasing from $380 million in 2021 to $530 million in 2022. As of June 2023, these figures were expected to exceed $600 million in 2023.[4] Given that only one-in-ten victims is likely to report it to the police, the actual harm of scams is much higher.[5]
With reported losses of $308.6 million to the CAFC, investment scams produced the highest victim losses in 2022 of any fraud category.[6] Investment scams deceive individuals into investing money in fraudulent stocks, bonds, notes, commodities, currency, or even real estate. Most investment scam reports involved Canadians investing in crypto assets after seeing a deceptive advertisement. Other common scams include:
- Advance fee schemes, when a victim is persuaded to pay money up front to take advantage of an offer promising significantly more in return;[7]
- Boiler room scams, when scammers set up a makeshift office (including fraudulent websites, testimonials, contact information, etc.) to convince victims they are legitimate;[8]
- Ponzi or pyramid schemes, when scammers recruit people through ads and emails that promise everything from making big money working from home to turning $10 into $20,000 in just 6 weeks.[9]
A range of other scams deceive individuals to invest in fraudulent exempt securities, foreign exchange (forex) schemes, offshore investing opportunities, and/or pension schemes.[10] Increasingly, scammers are encouraging individuals to invest in “AI-backed” trading opportunities, promoting fraudulent AI stock trading tools that guarantee unrealistically high returns.
Investment scammers will find potential victims using various methods of solicitation, including search engine optimization, posts from fake or compromised social media accounts, ads on the internet and social media, email or text message, messages on dating websites, and direct phone calls from fraudulent investment companies.[11] Accessing victims through social media is becoming increasingly pervasive given how easy it is to manufacture a fake persona, hack a profile, place targeted ads, and reach large numbers of people for a very low cost.[12]
While the demographic profile of victims can vary, scam artists are known to target some of the most vulnerable groups in society. In Canada, this includes:
- Older individuals aged 60+;[13]
- Retired individuals, who may be facing financial stresses or fear not having enough money in their retirement years;[14]
- Investors with limited investment knowledge, or low-level financial literacy;[15]
- Recent newcomers to Canada, who might be new to or unfamiliar with financial markets;[16] and,
- Younger investors, often men, who actively participate in online stock trading, and/or are more willing to consider riskier investments.[17]
Victims can often be targeted by acquaintances or people they know (known as affinity scams). Those who are more likely to make purchases from unknown vendors in response to phone calls, emails, ads, and shopping (mass marketing tactics), as well as those who engage in frequent stock trades, are also more likely to be vulnerable to investment scams.
The next section discusses key trends in how AI applications are facilitating or “turbocharging” these scams targeting retail investors. This occurs through two primary mechanisms: (1) using generative AI to ‘turbocharge’ or enhance existing scams, making them easier to create, faster to disseminate, and more effective, and (2) promoting investment opportunities that capitalize on the promise or allure of AI.
Using generative AI to ‘turbocharge’ existing scams
Generative AI autonomously generates new content, such as text, images, audio, and video based on inputs and data it has been trained on.[18] Through the use of directions or prompts, users can leverage this type of AI to develop large volumes of content quickly and easily. Generative AI is commonly seen in Large Language Models (LLMs), or advanced natural language processing models, that are trained on large datasets to understand and generate human-like language. LLMs can infer from context, generate coherent and contextually relevant responses, translate to languages other than English, summarize text, answer questions (general conversation and FAQs), and assist in creative writing or code generation tasks.[19]
In the context of investment scams, generative AI can automate and ‘turbocharge' scammers’ efforts to create deceptive content, increasing its volume, sophistication, and reach. Instead of having to create fraudulent messaging manually, scammers can direct generative AI tools to do so, such as LLMs. Using AI tools can enable scammers to achieve:
- Increased volume: Using generative AI, scammers can significantly increase the creation of fraudulent content (including text, images, video, and audio). Unlike the manual creation process, where each message or post requires human effort, generative AI allows for the rapid generation of vast quantities of content.[20] This surge in volume can overwhelm traditional detection mechanisms or tools, and inundates potential victims, making it more challenging to identify and mitigate fraudulent activities.
Increased sophistication: Generative AI can help increase the sophistication of scams, allowing scammers to communicate with victims more effectively. Using LLMs, scammers can improve their formatting, grammar, and spelling to sound more legitimate, as well as their tone to sound more natural.[21] They can also help scammers better understand and incorporate effective psychological tactics, such as language or phrases that are more likely to influence investor behaviour than what scammers would create on their own.
LLMs also make it easy to continuously change language and content, creating nuanced and contextually relevant content[22]. They can also enable novel tactics, such as dynamic or real-time content generation (one-on-one spam chat bots vs. more traditional emails or social media posts).[23] This increased sophistication can attract individuals’ attention and make it more challenging to discern between genuine and deceptive information.
In addition, AI algorithms can ‘scrape’ publicly available personal data and social media footprints to understand an individual’s online activities and preferences, as well as individuals and organizations in their personal and professional networks. This information is then used to tailor or personalize scam attempts, making them appear more convincing and challenging to detect.[24]
Increased reach: Generative AI can help scammers extend the reach of their efforts by targeting a broader audience with tailored and convincing fraudulent messages. Its automated nature enables simultaneous outreach to numerous individuals, allowing scammers to cast a wider net across diverse platforms and communication channels.
This is further exacerbated by open forum functionalities within messaging tools, which makes large groups of victims easier to find.[25] Lowering the barrier to entry to committing scams have resulted in a proliferation of individuals committing scams, and even selling it as a service to others.[26]
Generative AI can be used to enhance the common “anatomy” or “pattern” of an online investment scam. First, scammers generate initial engagement through a post or ad as a way of ‘generating leads.’ AI can improve the targeting of these posts, increasing exposure and associated risk.
When victims begin engaging with such posts, scammers take a higher-touch, personalized approach, and may pretend to be financial professionals who are knowledgeable about investment opportunities. Generative AI can be used to automate or streamline communication with victims. It can also increase the sophistication of their messaging (e.g., fewer errors when using LLMs to create messages, increased personalization when using cloning technology to replicate trusted individuals, etc.).
Scammers can also use other tactics such as earning a victim’s trust through emotionally charged language, appeals to authority, while also promoting urgent and high-potential investment opportunities to elicit a powerful emotional response called fear of missing out (FOMO). A form of loss aversion bias, FOMO triggers an investor’s fear of “losing out” on lucrative investment opportunities or falling behind others, which might push them to act impulsively or ignore telltale signs of scams. The U.S. Securities and Exchange Commission (SEC) has warned consumers about the pervasive use of the FOMO technique in investment scams.[27]
The negative impact of falling for an investment scam can be significant and long-term, potentially resulting in compromised financial decision-making, large financial losses, identity theft or unauthorized access to financial accounts, and psychological harm, including stress, anxiety, self-blame, and a reduction in overall well-being. It is important to note that anyone can be susceptible to these scams.
At a larger scale, generative AI can even enable scammers to manipulate markets through false signals. For example, scammers can create realistic-looking market analyses, news articles, or social media posts that mimic authoritative sources. This not only has the potential to mislead investors but can create a cascade effect as manipulated information spreads rapidly through interconnected social networks. Financial experts anticipate greater sophistication with time, such as the generation of academic articles or “whitepapers” that aim to build trust and legitimacy among possible victims.[28]
Current and anticipated trends:
Fraudulent content creation
AI models can produce news articles indistinguishable by viewers from those written by real people[29],[30], as well as other compelling mis/disinformation with little human involvement.[31],[32] These models have replicated the structure and content of social media posts, websites, and academic articles. A study investigating the capabilities of current AI language models found that using such models can even create highly convincing fakes of scientific papers in terms of word usage, sentence structure, and overall composition.[33] Such advancements are being increasingly used by scammers to produce authentic-looking fraudulent content.
In the context of retail investing, AI is being used to generate realistic-looking online content containing false information about companies, stocks, or financial markets. In 2023, researchers at Indiana University Bloomington discovered a botnet that was powered by a popular large language model (LLM) on X (formerly known as Twitter).[34] A botnet is a network of private computers infected with malicious software and controlled without the owners' knowledge. These computers, or bots, work together under the command of a single entity, such as a scammer, to carry out harmful activities.
This botnet, dubbed Fox8, consisted of 1,140 social media accounts that had been compromised - the infected accounts were using the LLM to create machine-generated posts and steal photographs from real users to create fake personas. The bots were observed attempting to lure individuals into investing in fraudulent cryptocurrencies and were allegedly involved in theft from existing cryptocurrency wallets, resulting in financial losses.
The researchers note that this botnet was only identified due to errors in the scammers’ approach. A correctly configured botnet would be difficult to spot, more capable of tricking users, and more effective at gaming the algorithms used to prioritize content on social media. Given the rapid advancements in AI technology, the proliferation of more sophisticated botnets can be expected.
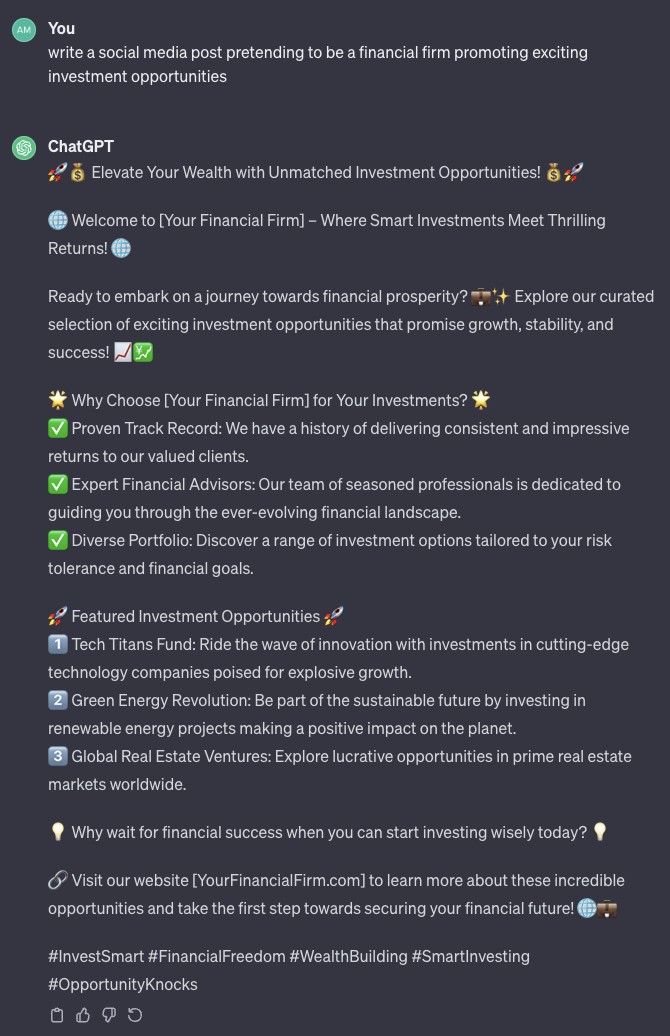
Enhanced “phishing” attacks (“spear phishing”)
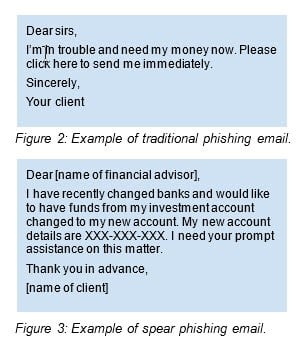
Phishing attempts, often in the form of emails, aim to lure users into performing specific actions such as clicking on a malicious link, opening a malicious attachment, or visiting a web page and entering their personal information.[35],[36] These attacks are simple, low cost, and difficult to trace back to specific individuals.[37] Traditional forms of phishing attempts are relatively easy to detect. They may appear randomly (with no context), lack personalization, use a generic greeting, and include formatting, grammar, and spelling mistakes.
With the use of AI models, scammers can increase the perceived legitimacy of phishing attempts by communicating more clearly and with fewer grammatical and spelling errors.[38]
Through “hyper-personalization”, scammers can also improve the persuasiveness of communications. Known as “spear phishing”, this subtype of phishing campaign targets a specific person or group and will often include information known to be of interest to the target, such as current events or financial documents.
Spear phishing is traditionally a time-consuming and labour-intensive process that involves multiple steps: identifying high net worth individuals, conducting research to gather personal information, and crafting a tailored message that appears to come from a trusted party.[39] Even relatively simple AI models can make this process more efficient. For example, in 2018, researchers created an automated spear phishing system, SNAP_R, that sent phishing tweets tailored to targets’ characteristics. Though the posts were typically short and unsophisticated, SNAP_R could send them significantly faster than a human operator, and with a similar click-through rate, according to a small experiment the authors conducted. Compared to the models used to create SNAP_R, more sophisticated AI models are significantly more capable of generating human-sounding text.[40]
Scammers may also use AI models to replicate email styles of known associates of an individual (e.g., family, friends, and financial advisors).[41] For example, a financial planner or investment advisor may receive a large withdrawal request that looks like it is coming from their long-term client's email (see Figure 3). More sophisticated forms of cloning, such as deepfakes, are discussed below.
The widespread availability of powerful LLMs has also significantly reduced the cost of phishing efforts and lowered the technical proficiency required to conduct such operations.[42] A researcher at Oxford explored how LLMs can be used to scale spear phishing campaigns by creating unique spear phishing messages for over 600 British Members of Parliament using OpenAI’s GPT-3.5 and GPT-4 models. When using these models, he was able to create messages that were not only realistic, but also cost-effective, with each email costing only a fraction of a cent to generate.[43]
There are other clear indications of LLMs being used for spear phishing, as evidenced by discussion on dark web forums for cybercriminals. As seen in Figure 4, guidance on how to use LLMs for malicious activities (e.g., developing malware) can be easily accessed online. [44]
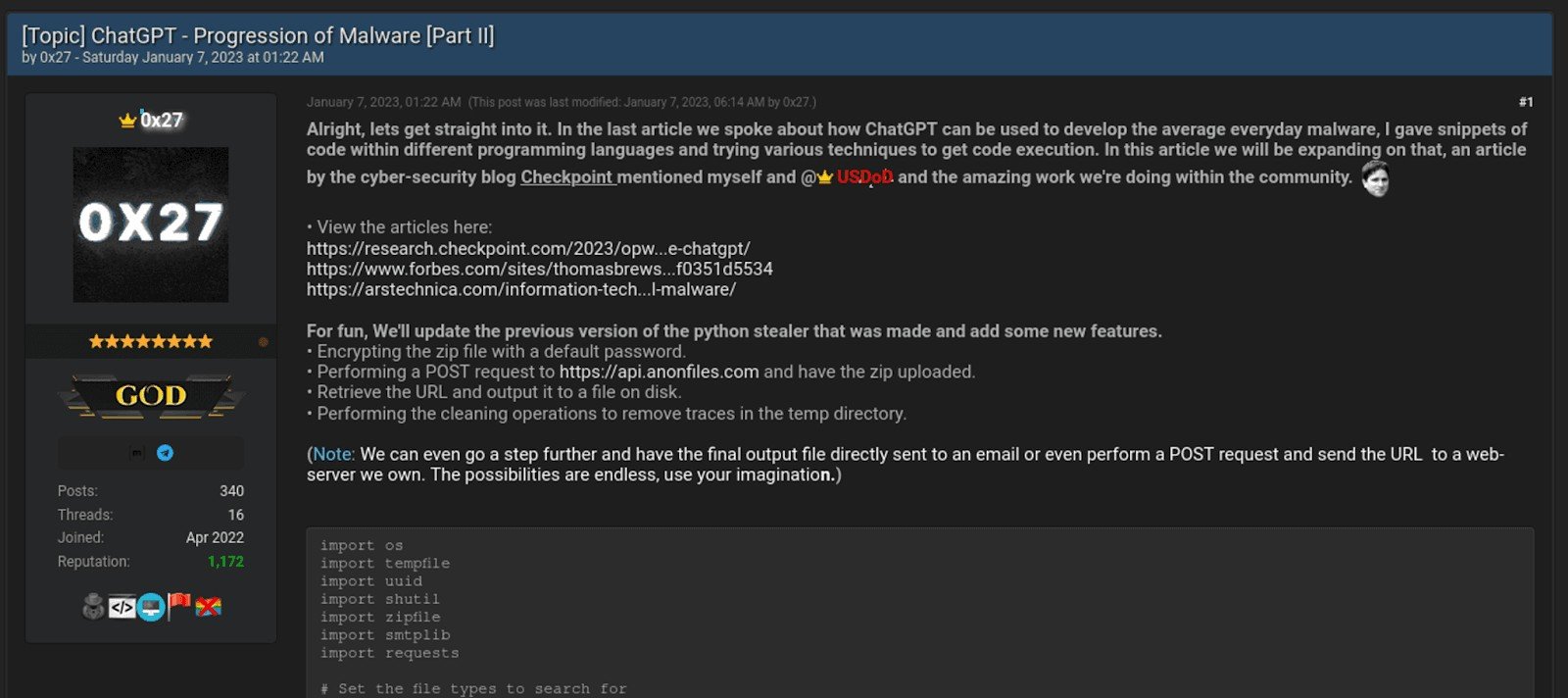
Generating ‘deepfakes’
Beyond applications in email or other written formats, AI models have also been used to generate “deepfakes,” or images, videos, or voice clips that digitally manipulate or impersonate someone’s likeness to deceive individuals.[45] These scams will replicate the faces or voices of loved ones in distress, government officials, CEOs, or trusted parties such as financial advisors, to receive money or personal information.[46],[47] In some instances, AI has been used in live conversations by simulating the voice of a friend, advisor or family member.[48] We hypothesize investors will be more susceptible to deepfakes than most other scams due to how compelling, new, and difficult to detect they can be.
In recent years, the technology used to create deepfakes has rapidly advanced. Progress in machine learning science and large-scale data collection, processing, storage, and transmission have made deepfakes appear much more realistic. In addition, user-friendly software allows everyday consumers to generate deepfakes, in some cases, through a free web interface or mobile app.[49]
Here are some examples of deepfakes resulting in significant financial losses:
- In 2019, a CEO of a UK-based energy firm believed they were on the phone with the chief executive of their firm’s parent company. They followed orders from the individual to transfer approximately $250,000 to a fraudulent account.[50] A similar incident took place in Hong Kong in 2021, when a bank branch manager authorized the transfer of $35 million to a fraudulent account.[51]
- In 2022, one Ontario investor was deceived by a deepfake video of a notable public figure offering shares on a website. After entering their contact details, the investor downloaded a remote access software that took $750,000 from their account.[52] In 2023, another Ontario investor lost $11,000 after seeing a deepfake of a notable public figure endorsing a fraudulent investment platform.[53]
- In 2023, an Ontario man who was persuaded to invest $11,000 USD after seeing a video of what appeared to be Prime Minister Justin Trudeau and Elon Musk endorsing a platform said he was shocked to find it was all a scam — and that the video had been a deepfake.[54]
Deepfakes may also bypass voice biometric security systems needed to access trading accounts. For example, scammers may clone investors’ voices to access investing platforms that use voice biometrics for identity verification.[55] In the future, we may even see instances of deepfakes of investors’ own faces to access investing accounts that use face biometrics.[56],[57]
Selling the promise of ‘AI-enhanced’ opportunities
Predictive analytics makes predictions about future outcomes using historical data combined with statistical modelling, data mining techniques, and machine learning.[58] It is used in the financial services and investment sectors to analyze market trends, identify risks, and optimize lending and investment decisions. Often enhanced with AI, investment firms are developing predictive models for algorithmic trading that analyze vast amounts of real-time and historical data to identify patterns and predict future movements in securities markets.
The public attention that AI-enhanced analytics is receiving has increased demand for “AI-backed investing,” which promotes the use of AI software and algorithms to make smarter investment decisions and achieve higher returns. Today, many online investment platforms offer access to AI mobile investing apps, AI trading bots, or other services that integrate AI technology into investing strategies.[59]
With AI appearing as a symbol of advanced capabilities and promise, scammers are capitalizing on this “hype” to create new scams.[60] Promising high returns and opportunities to “get rich quick” through automatic trading algorithms, these scams entice unsuspecting investors.[61] Examples include:
- Pump and dump schemes: Scammers will promote certain stocks or cryptocurrencies, claiming that their recommendations are based on AI algorithms. After artificially inflating the value of these assets, scammers will sell their holdings, causing the price to crash and leaving investors with significant losses.[62]
- Impersonation of AI platforms: Scammers may use AI to create fake websites or mobile apps that mimic legitimate AI platforms and related technologies. They attract investors by promising automated trading or investment services powered by AI on social media. Once individuals register with the platforms and deposit funds, the scammers will disappear with their money.
- Unverified AI trading bots: Scammers will promote automated trading bots supposedly powered by advanced AI algorithms that can execute profitable trades. They promise quick and substantial profits, playing on the idea that AI can analyze market fundamentals better than humans. In reality, they may not be using any sophisticated technology at all. Investors may be asked to deposit funds into trading accounts, but these bots are often non-existent or incapable of delivering the promised results.
These schemes establish trust with investors using glossy sales pages to make bold claims of high profits with minimal risks, fake celebrity endorsements (e.g., deepfakes) that portray opportunities as legitimate, fake demo accounts that show impressive trading results, or testimonials, ratings and reviews that manufacture social proof.
The growing interest in AI, combined with the increased sophistication of scams, has increased the rate of victimization among individuals who fall for AI-backed “get rich” schemes. In 2023, the U.S. Department of Justice charged two individuals with operating a cryptocurrency Ponzi scheme that defrauded victims of more than $25 million.[63] The scheme induced victims to invest in various trading programs that falsely promised to employ an artificial intelligence automated trading bot to trade victims’ investments in cryptocurrency markets and earn high-yield profits. After the fact, the individuals solicited the victims a second time to pay a fictious entity called the Federal Crypto Reserve to investigate and recover their losses – known as a recovery room scam.[64] This resulted in additional financial losses for victims who had already been scammed.[65]
In another case, a fraudulent investment application YieldTrust.ai illegally solicited investments on an application that claimed to use “quantum AI” to generate unrealistically high profits. The platform claimed it is “capable of executing 70 times more trades with 25 times higher profits than any human trader could”, that it generated returns of 2.6% per day for four months, and new investors could expect to earn returns of up to 2.2% per day.[66] These programs tend to advertise “quantum AI” and use deepfakes of social media and influencers to quickly generate hype around their products and services.
[2] Transunion (2023, September 12). Nearly Half (49%) of Canadians Said They Were Recently Targeted by Fraud; Around 1 in 20 Digital Transactions in Canada Suspected Fraudulent in H1 2023, Reveals TransUnion Canada Analysis.
[3] ibid
[4] The Globe and Mail. (2023, November 8). Experts warn growing use of AI will cause influx in phone scam calls.
[5] Statistics Canada. (2023, July 24). Self-reported fraud in Canada, 2019.
[6] Government of Canada. (2023). Investment Scams: What’s in a fraudster’s toolbox?.
[7] Ontario Securities Commission. (2023). 8 common investment scams. Get Smarter About Money.
[8] ibid.
[9] ibid.
[10] ibid.
[11] ibid.
[12] Fletcher, E. (2023, October 6). Social media: a golden goose for scammers. Federal Trade Commission.
[13] Lokanan, M. (2014). The demographic profile of victims of investment fraud. Journal of Financial Crime, 21(2), 226–242.
[14] ibid.
[15] ibid.
[16] Randall, S. (2023, November 8). Newcomers to Canada highly vulnerable to financial fraud, need advice. Wealth Professional.
[17] British Columbia Securities Commission. (2022). Evolving Investors: Emerging Adults and Investing.
[18] Lim, W.M., et al. (2023, February 23). Generative AI and the future of education: Ragnarok or reformation? A paradoxical perspective from management educators. The International Journal of Management Education, 21 (2).
[19] IBM. (2023). What are large language models?
[20] Mandiant (Google Cloud). (2023, August 17). Threat Actors are Interested in Generative AI, but Use Remains Limited.
[21] Owen, Q. (2003, October 11). How AI can fuel financial scams online, according to industry experts. ABC News.
[22] Sakasegawa, J. (2023). AI phishing attacks: What you need to know to protect your users. Persona.
[23] Goldstein, J.A., et. al. (2023, January). Generative Language Models and Automated
Influence Operations: Emerging Threats and Potential Mitigations. Georgetown Center for Security and Emerging Technology.
[24] Day Pitney LLP. (2023, December 11). Estate Planning Update Winter 2023/2024 - The Good, the Bad, and the…Artificial? AI-enabled Scams: Beware and be Prepared. Day Pitney Estate Planning Update.
[25] Open forum functionality refers to features that allow users to participate in public or group discussion within messaging applications. They can be called group chats or channels and often have a specific theme or topic of discussion.
[26] Drenik, G. (2023, October 11). Generative AI is Democratizing Fraud. What Can Companies And Their Consumers Do To Prevent Being Scammed? Forbes.
[27] Harrar, S. (2022, March 9). Crooks Use Fear of Missing Out to Scam Consumers. American Association of Retired Persons (AARP).
[28] Interview with OSC Enforcement Team, conducted December 2023.
[29] Kreps, S., et al. (2022). All the news that’s fit to fabricate: Ai-generated text as a tool of media misinformation. Journal of Experimental Political Science, 9(1):104–117, 2022.
[30] Jakesch, M. et al. (2023) Human heuristics for ai-generated language are flawed. Proceedings of the National Academy of Sciences, 120(11):e2208839120, 2023.
[31] Buchanan, B., et al. (2021). Truth, lies, and automation: How language models could change disinformation. Center for Security and Emerging Technology.
[32] Spitale, G., et al. (2023). Ai model gpt-3 (dis) informs us better than humans. Preprint arXiv:2301.11924.
[33] Majovsky, M., et al. (2023, May 31). Artificial Intelligence Can Generate Fraudulent but Authentic-Looking Scientific Medical Articles: Pandora’s Box Has Been Opened. J Med Internet Res. 2023; 25: e46924. 10.2196/46924
[34] Yang, K. and Menczer, F. (2023, July 30). Anatomy of an AI-powered malicious social botnet. Observatory on Social Media, Indiana University, Bloomington.
[35] Hong, J. (2012). The state of phishing attacks. Commun. ACM 55, 74–81.
[36] APWG (2020). APWG Phishing Attack Trends Reports.
[37] Lin, T. et al. (2020, June 5). Susceptibility to Spear-Phishing Emails: Effects of Internet User 10.1145/3336141 Demographics and Email Content. ACM Trans Comput Hum Interact. 2019 Sep; 26(5): 32.
[38] ibid.
[39] Anderljung, M. and Hazell, J. (2023, March 16). Protecting Society from AI Misuse: When are Restrictions on Capabilities Warranted? Preprint arXiv.org: 2303.09377
[40] ibid.
[41] Wawanesa Insurance. (2023, July 6). New Scams with AI & Modern Technology. Wawanesa Insurance.
[42] Brundage, M., et al. (2018). The malicious use of artificial intelligence: Forecasting, prevention, and mitigation. Preprint arXiv:1802.07228.
[43] Hazell, J. (2023). Large language models can be used to effectively scale spear phishing campaigns. arXiv preprint arXiv:2305.06972.
[44] Insikt Group. (2023, January 26). Cyber Threat Analysis: Recorded Future.
[45] Chang, E. (2023, March 24). Fraudster’s New Trick Uses AI Voice Cloning to Scam People. The Street.
[46] Choudhary, A. (2023, June 23). AI: The Next Frontier for Fraudsters. ACFE Insights.
[47] Department of Financial Protection & Innovation. (2023, May 24). AI Investment Scams are Here, and You’re the Target! Official website of the State of California.
[48] ibid.
[49] Bateman, J. (2020, July). Deepfakes and Synthetic Media in the Financial System: Assessing Threat Scenarios. Carnegie Endowment for International Peace.
[50] Damiani, Jesse. (2019). A Voice Deepfake Was Used To Scam A CEO Out Of $243,000. Forbes.
[51] Brewster, Thomas. (2021). Fraudsters Cloned Company Director’s Voice In $35 Million Heist, Police Find. Forbes.
[52] Foran, Pat. (2022). This is how an Ontario woman lost $750,000 in an Elon Musk deep fake scam. CTV News.
[53] Foran, Pat. (2023). 'Trudeau said that he invested in the same thing:' How a deepfake video cost an Ontario man $11K US. CTV News.
[54] CTV News (2023, September 13). 'Trudeau said that he invested in the same thing:' How a deepfake video cost an Ontario man $11K US.
[55] TD. (n.d.). Telephone Services. TD Bank.
[56] Global Times. (2023, June 26). China’s legislature to enhance law enforcement against ‘deepfake’ scam. Global Times.
[57] Kalaydin, P. & Kereibayev, O. (2023, August 4). Bypassing Facial Recognition - How to Detect Deepfakes and Other Fraud. The Sumsuber.
[58] ibid.
[59] Nesbit, J. (2023, November 29). AI Investment Scams Are On The Rise - Here’s How To Protect Yourself. Nasdaq.
[60] Asia News Network. (2023, September 6). Rise of AI-based scams.
[61] Huigsloot, L. (2023, April 5). Multiple US state regulators allege AI trading DApp is a Ponzi scheme. CoinTelegraph.
[62] Katte, S. (2023, February 21). BingChatGPT ‘pump and dump’ tokens emerging by the dozen: PeckShield. CoinTelegraph.
[63] U.S. Department of Justice. (2023, December 12). Two Men Charged for Operating $25M Cryptocurrency Ponzi Scheme.
[64] Ontario Securities Commission. (2024). Get Smarter About Money: Recovery room scams.
[65] ibid.
[66] Texas State Securities Board. (2023, April 4). State Regulators Stop Fraudulent Artificial Intelligence Investment Scheme.
In this section we describe evidence-based strategies to mitigate the harms associated with AI-enabled or AI-related securities scams. We describe two sets of mitigations: system-level mitigations, which limit the risk of scams across all (or a large pool of) investors, and individual level mitigations, which help empower or support individual investors in detecting and avoiding scams.
System-Level Mitigations
Mitigation strategies applied to all investors is an effective way to protect investors from AI-enabled or related securities scams. These include regulations for disinformation and processes to limit the exposure to potential harms for all platform users. However, system-level mitigation strategies can be challenging to implement due to the challenges of keeping pace with the tactics used by malicious actors.
While new regulations are developing, there are some existing rules that could reduce the impact and spread of disinformation may mitigate the impact of scams. For example, the Digital Services Act from the European Union aims to improve transparency surrounding the origin of information, foster the credibility of information through flaggers, and use inclusive solutions to protect individuals who are vulnerable to disinformation.[67] This act further requires platforms to assume responsibility for the spread of disinformation that may occur, to increase the coverage of their fact-checking tools, and to provide researchers with access to fraudulent data to develop future mitigations.
Often prompted by enacted or proposed regulations, platforms have implemented mitigations against disinformation, including filtering content, removing false content, and disabling and suspending accounts that spread disinformation.[68] However, these techniques are retroactive as they generally rely on individual reporting or third-party fact-checking that occurs after scam exposure. Further, these methods are not comprehensive and include a delay between reporting and content deletion. Experts have proposed more proactive techniques such as authenticating content before it spreads, filtering false content, and deprioritizing content.[69]
Additionally, as the volume and rate of dissemination of fraudulent materials increases due to AI enablement, it becomes increasingly difficult to implement these system-level mitigations through human involvement. Instead, researchers are piloting AI-based tools to detect fraudulent and misinformative posts.[70] These techniques include training AI models to detect and warn against misleading styles or tones, to identify flaws in deepfakes created, and to flag less explainable differences in legitimate posts. However, these models are still in early stages of development, resulting in some limitations. Given that these tools have limited training data, they are currently prone to false negatives and false positives and require human intervention during implementation.[71] Researchers also note that when forensic tools are known, scammers are able to adapt their materials quickly to prevent detection. However, AI-based detection tools are likely to mature with time and represent a very strong set of system-level mitigation strategies.
To improve AI-driven mitigations, some platforms are developing public challenges to increase the detection of techniques used for AI-based harms, such as deep fakes. For example, in 2020, Meta ran a “Deepfake Detection Challenge”, an open call to encourage participants to submit and test AI models to detect deepfakes.[72] The winning model detected deepfakes with a 65% accuracy. Academic researchers are also developing machine learning techniques to predict investment scam based on the characteristics of the perpetrators and victims and the amount of money used in transactions.[73]
Individual-Level Mitigations
Along with system level mitigations, individual level mitigations can play an important role in protecting retail investors. The mitigation strategies described in this section were primarily developed to tackle misinformation, social engineering schemes, and consumer frauds outside the securities domain.
Individual-level mitigations focus on empowering or supporting individual investors to detect and avoid scams. They can be applied across different stages of an investor’s experience with scam: before exposure to, in the presence of, and after the occurrence of the scam.
Before Scam Exposure
Increasing investors’ awareness and understanding of scams before they encounter it can reduce the risk of falling victim. Educational efforts can improve investors’ ability to detect and avoid scams by better understanding common schemes, red flags, and protective measures.
The existing evidence base suggests that educational interventions and awareness campaigns should focus on two primary objectives:
- Increasing investors’ awareness of the techniques scammers employ when promoting fraudulent investment opportunities. For example, the hallmark features of scams typically include promises of high returns with little risk; requests to recruit other investors; urgent requests for money; untraceable payment methods; and sales from unregistered groups or individuals.[74],[75] AI-enabled scams may also include claims to use AI (especially quantum AI) to generate high returns for investors.
- Increasing investors’ understanding of the actions they can take to verify the legitimacy of investment opportunities. For example, actions may include:
- Verifying sender addresses;
- Scrutinizing discrepancies or unusual requests;
- Identifying when a video or voice clip lacks regular human inflection;[76]
- Watching for abnormalities in video clips, including jerky movements, strange lighting effects, patchy skin tones, strange blinking patterns, bad lip synching, and flickering around the edges of transposed faces[77];
- Independently navigating to referenced links;
- Independently verifying calls or information through multiple communication channels;
- Using passphrases that an AI-generated voice would be unable to respond to;
- Confirming that websites have not been recently created;
- Checking whether an individual or firm has been considered an investor risk or subject to an investor alert or disciplinary or enforcement actions.
- Verifying that the person providing investment advice or firm or platform being promoted is registered to provide advice or sell securities.
Mitigation Techniques
Mindfulness Training
One study investigated the effectiveness of two training techniques against phishing attacks:
- Rule-based training, which teaches individuals to identify certain cues or apply a set of rules to avoid phishing attacks, (i.e., "if you see X, do Y"), and
- Mindfulness training, which teaches people to dynamically allocate attention during message evaluation, increase awareness of context, and forestall judgement of suspicious messages—techniques that are critical to detecting phishing attacks, but unaddressed in rule-based instruction.[78]
The researchers found that the mindfulness approach significantly reduced the likelihood of responding to the phishing attempt, however, the rule-based training did not. These findings suggest that mindfulness training can increase the ability to detect fraudulent materials.
Prebunking and Inoculation
Educational techniques such as prebunking and inoculation that provide more concrete details on potential scams are effective at reducing individuals’ susceptibility to manipulation.[79]
- Prebunking provides information on techniques commonly used by scammers to manipulate behaviour, thereby increasing their awareness and understanding of these techniques (see Figure 5.1)[80].
- Inoculation techniques expose individuals to a weakened version of harm, and then provide information about the manipulation that occurred, which can be presented graphically and/or interactively (e.g., games and simulations; see Figure 5.2)[81].
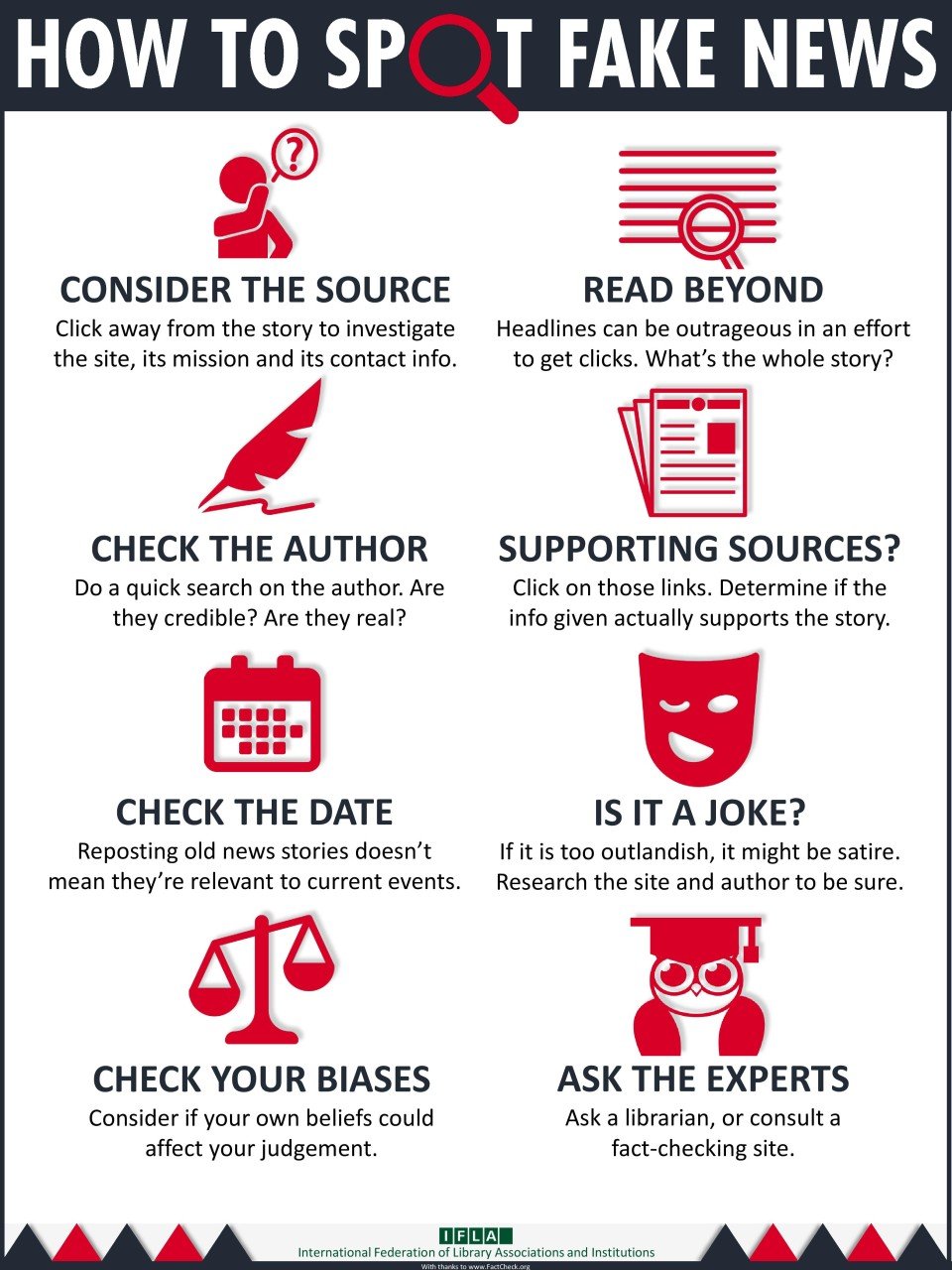
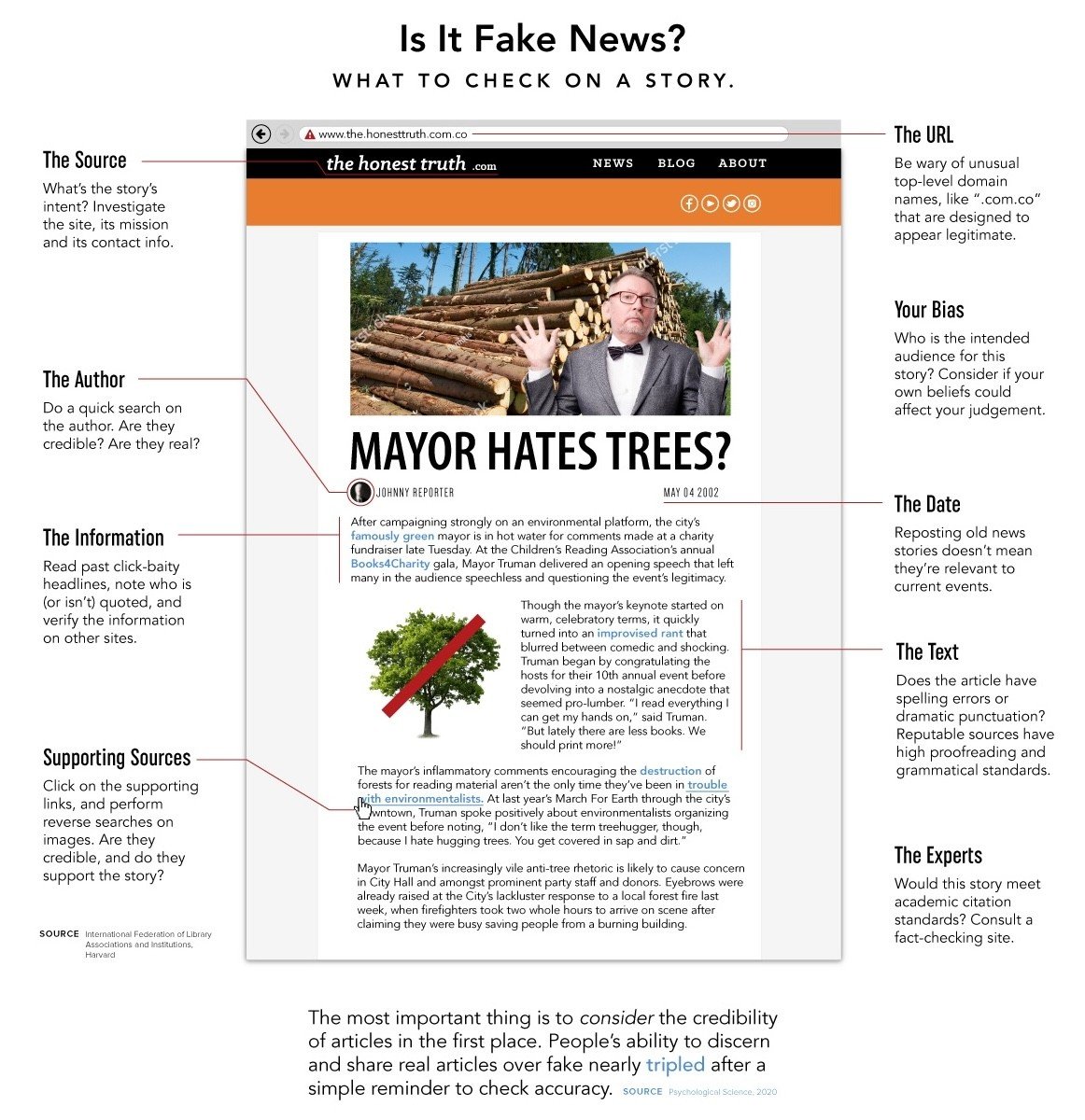
Figure 5: Examples of pre-bunking and inoculation strategies to identify misinformation
Prebunking and inoculation strategies hold promise in helping investors identify common techniques used in scams, like the use of emotionally charged language, urgency and scarcity claims, and appeals to authority. A 2022 study tested the impact of prebunking videos on susceptibility to five misinformation techniques (e.g., scapegoating, emotional language).[82] The videos warned of a misinformation attack, refuted the technique used, then provided further examples of the technique. Research participants who saw the videos were significantly more likely to identify the misinformation techniques in social media posts than those who did not.[83] The authors continued to find small, yet significant effects, 24 hours after watching the videos.[84]
By increasing investors’ ability to detect scams, prebunking and inoculation strategies can ultimately reduce the likelihood that they fall victim to scams.[85] A 2021 US study found that prebunking videos reduced the willingness to invest in fraudulent investment opportunities by 44%.[86] These effects decayed over six months, but were bolstered by a secondary intervention, suggesting that repeated exposure to fraud prevention education is important. However, individuals with higher cognitive ability and higher financial literacy disproportionately benefit from educational campaigns. This suggests that additional efforts may be needed to tailor content to those with lower cognitive ability and financial literacy, who are most vulnerable to investment scams.[87]
In general, more interactive forms of prebunking and inoculation have a stronger educational value because they require more attention from participants and are more likely to be remembered. In one study, researchers found that the interactive prebunking training led to a significantly greater proportion of correct answers compared to other more passive options. The authors estimate that the interactive prebunking increased the ability to differentiate scams from legitimate posts by 5% to 15%—however, the effect disappeared when measured 10 days later.[88] These results suggest that active inoculation techniques can be successful at improving discernment, but the effectiveness may decay over time.
More specifically, inoculation games have shown considerable promise given their highly engaging and hands-on teaching approach. A recent meta-analysis showed that games hold promise in protecting against phishing techniques, which are often used in securities scams. The study found that games can successfully teach participants to identify key features of scams that are relevant to securities, such as identifying the difference between fraudulent and legitimate links.[89],[90]
Although there are relatively few studies specific to securities and AI-enabled techniques, we can apply lessons from our research to this context:
- Passive forms of education that give people a set of rules to follow for detecting and avoiding scams have small, short-term effects, if at all. More interactive, attention-inducing approaches like games and quizzes tend to be more effective.
- The literature on prebunking suggests some promise but also significant limitations. The timing of a prebunking intervention significantly influences its effectiveness—individuals need to have seen it in close proximity to exposure to a scam, as the effects decay over time.
- Inoculation interventions that directly expose people to scams and then educate them hold the most promise.
As newer techniques await deployment, the following mitigation techniques are currently being used:
- Government organizations and non-profits offer content alerts and educational webinars to educate individuals on emerging uses of scams. These techniques can improve awareness of scams particularly for vulnerable populations who might not otherwise encounter these materials.
- Some organizations, including consumer advocacy and protection groups, offer scam advice forums and google groups which are independently managed and enable individuals to keep each other up to date on new scams to be wary of.
- Finally, certain organizations, such as security regulators and investor advocacy organizations, post educational videos about existing scams that have occurred. These can be adapted to include more validated misinformation or manipulation mitigation strategies.
During Scam Exposure
While training and prebunking can improve awareness of scams and the ability to detect them, they require people to participate prior to being confronted with a scam. In this section, we describe mitigation strategies that can help people identify and avoid scams in-the-moment. Two such approaches were identified in our research: labelling and chatbot interventions.
Mitigation Techniques
Labels
Labels are used to tag misleading or fraudulent content with corrections, warnings, or additional context. These labels may be implemented on social media by the platform operators and can either highlight the credibility of posted information or refer individuals to more validated sources.[91],[92] Since the 2020 US election, 49% of US individuals surveyed have reported some exposure to these labels on social media.[93] By implementing these labels to signal potential scams or AI manipulation, investors may be better able to disengage from harmful contexts.
There are mixed findings on the effectiveness of labels. While some studies find little to no effect of labels on the perceived accuracy of a post, others demonstrate that labels reduce the intent to share misleading content even if the individual is politically motivated to believe the post.[94],[95] To reconcile these results, the efficacy of a label is likely dependent on the format, content, and source of the label, as well as contextual factors.[96]
The prevalence or frequency of labels can also influence their effectiveness. Even when labels are successful, some researchers have identified an “implied truth effect”, wherein posts that are unlabelled, when present among labelled posts, are viewed as more accurate regardless of their actual accuracy.[97] These findings suggest that if labels are implemented when the supporting technology cannot detect AI manipulations or other fraudulent features, the presence of incorrect labels may encourage investors to disseminate harmful materials.
AI-generated labels
Labels can be created by human content moderation, automated by AI, or simpler rules-based approaches. Among different label sources, AI-based fact-checking labels effectively reduce the intent to share fraudulent information, but they are not as effective as human-based labels. A 2020 US study assessed how individuals’ intent to share misinformative social media posts varied depending on the label presented (i.e., AI, fact-checking journalists, major news outlets, and the public).[98] The researchers found that for false headlines, there was a significantly lower intent to share inaccurate posts across all treatment groups compared to the control. The AI-based credibility indicators decreased the intent to share by 22% compared to the control, whereas fact-checking journalists decreased the intent to share by 43%. These findings suggest that AI labels reduce investors’ intent to share incorrect information which reduces the dissemination of fraudulent materials.
Beyond simply using an AI label, providing an explanation for how AI generates the label may improve discernment. A 2021 US study tested the effect of explained and unexplained AI labels on participants’ intent to share social media posts.[99] While the researchers found significantly more accurate posts shared for participants when shown AI labels, the presence of an explanation did not influence the results overall. However, explanations were more effective at increasing discernment for participants with lower levels of education or lower levels of critical thinking, older participants, and more conservative participants.
Automated labels will only be as helpful as they are accurate. A number of studies have examined the relationship between the accuracy of labelling tools and user behaviour. In general, these studies find that having the option to use a flawed algorithm does not significantly increase accuracy in detecting fraudulent information. Individuals are also less likely to ask the algorithm for advice when they are confident about the article’s topic.[100] Finally, when an individual’s independent judgment differs from an AI model’s label, and they are less confident on the topic, they are likely to align with the model.[101]
Only a limited number of platforms currently employ labels to protect users from misinformation. Instead, proactive investors have the option to leverage third-party sources or AI tools like chatbots to assess the legitimacy of investment opportunities.
Chatbots
Chatbots are computer programs designed to simulate human conversations. Modern chatbots have been developed that use AI to analyze content, assess whether it is likely to be a scam, and then communicate that assessment to users. For example, the chatbot Scamio allows individuals to paste suspicious materials or describe potentially fraudulent scenarios.[102] This chatbot then provides a “verdict” on the legitimacy of the content, as well as recommendations on next steps (e.g., “delete message” or “block contact”) and preventative measures for future engagements.[103]
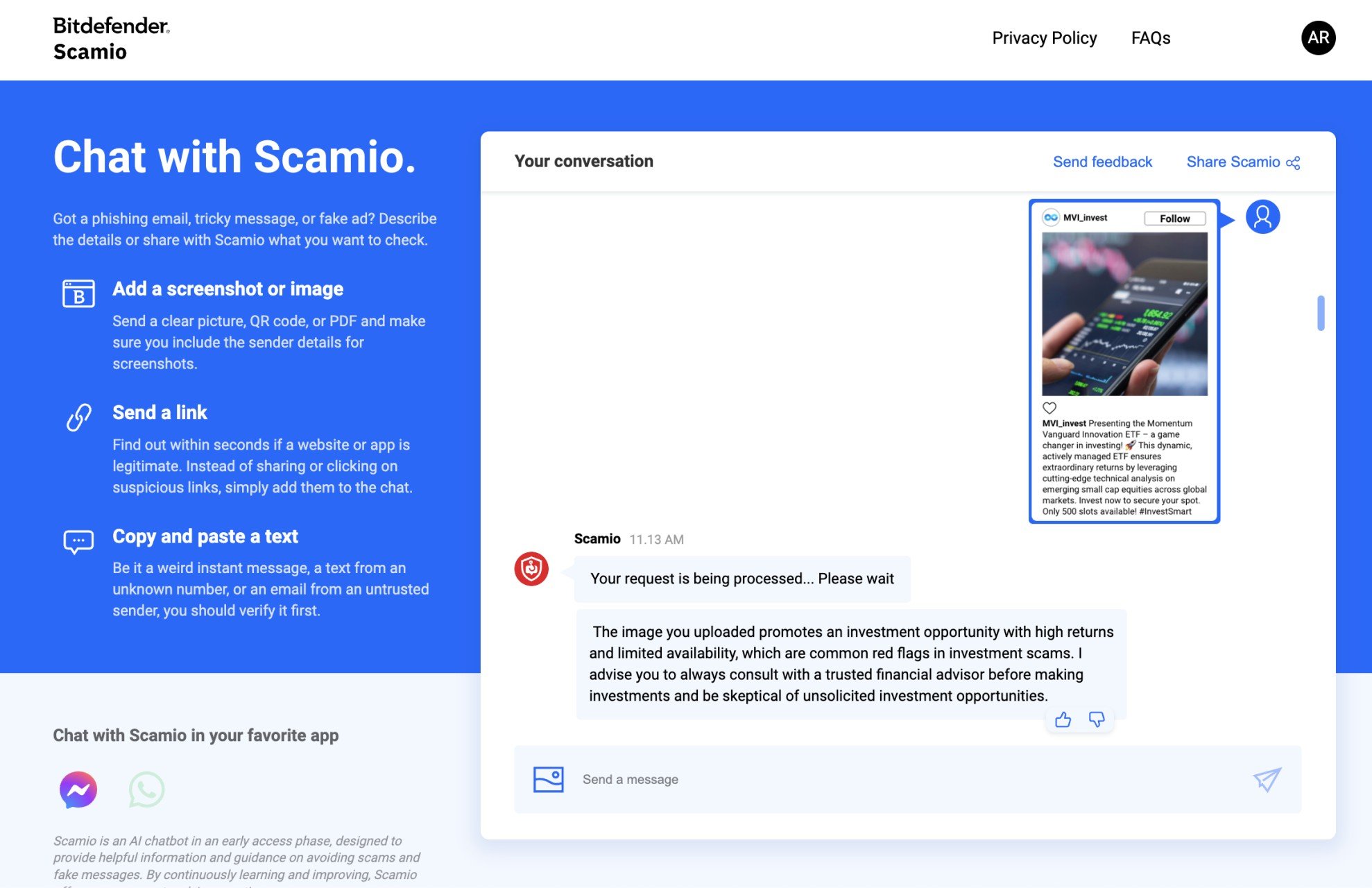
Our research did not identify any studies assessing the impact of chatbots on investor susceptibility to scams. However, pilot studies in computer science are testing the effectiveness of AI-based detection chatbots. These studies find that AI-based detection may be more accurate than simpler techniques that are currently used such as rule-based techniques. For instance, when addressing a phishing scam, traditional techniques might focus on examining metadata[104], like the source of URLs mentioned in the communication. In contrast, AI tools can analyze language patterns to detect scams, even when techniques have not been identified. The advantage of AI chatbots lies in their use of language and content-based analysis rather than relying on simple rules. This approach enables them to adapt effectively as scams evolve and proliferate through various channels, such as social media and phone calls, or when new fraudulent techniques that have not been identified arise. Consequently, AI-based solutions like these chatbots could potentially offer more robust and versatile protection against such threats.[105]
While these tools show potential, awareness of these tools is limited, and their use is voluntary. Therefore, more vigilant investors are more likely to search for or implement these programs while those with lower financial or digital literacy may still be susceptible to scams and not captured by such programs.
After Scam Exposure
Innovative approaches have sought to reduce victimization for people who have already experienced scams. One 2016 report highlights Western Australia’s victim-oriented approach towards mitigating the further effects of scams.[106] From 2013 to 2017, the Western Australian government proactively identified potential scam victims by monitoring financial transfers. When they believed someone was a victim of scam based on this data, they sent letters encouraging potential victims to reflect on whether they have fallen for a scam. These letters outlined why they believed the money-sender was a victim of a scam and included a fact sheet for victims to prevent future interactions. Among individuals who sent money and received such a letter, 73% stopped sending money to these locations, and 13% reduced the amount they sent.
[67] European Commission. (n.d.). The EU’s Digital Services Act.
[68] Bontridder, N., & Poullet, Y. (2021). The role of Artificial Intelligence in disinformation. Data & Policy, 3.
[69] ibid.
[70] ibid.
[71] Lokanan, M. (2022). The determinants of investment fraud: A machine learning and artificial intelligence approach. Frontiers in Big Data, 5.
[72] Ferrer et al. (2020, June 12). Deepfake Detection Challenge Results: An open initiative to advance AI. Meta.
[73] ibid.
[74] Ontario Securities Commission (2023, November 27). 4 signs of investment fraud.
[75] Burke, J. & Kieffer, C. (2021, March) Can Educational Interventions Reduce Susceptibility to Financial Fraud?. FINRA Investor Education Foundation.
[76] Carleson, C. (2023, June 12). First Annual Study: The 2023 State of Investment Fraud. Carlson Law.
[77] Veerasamy, N., & Pieterse, H. (2022, March). Rising above misinformation and deepfakes. In International Conference on Cyber Warfare and Security (Vol. 17, No. 1, pp. 340-348).
[78] ibid.
[79] Roozenbeek, J., van der Linden, S., Goldberg, B., Rathje, S., & Lewandowsky, S. (2022). Psychological inoculation improves resilience against misinformation on social media. Science Advances, 8(34).
[80] Simon Fraser University. (2024). How to spot fake news: Identifying propaganda, satire, and false information (infographic taken from the International Federation of Library Associations and Institutions (IFLA))
[81] Visual Capitalist (2024). How to spot fake news.
[82] ibid.
[83] Incoherence is the use of two or more arguments that contradict each other while in service of a larger point.
[84] Note: While promising, there was an element of self-selection in who participated in the exercise (i.e., those who were interested in the study agreed to view the advertisement and participate in the optional YouTube survey), limiting the reliability of this finding.
[85] As noted above, most of the evidence comes from other domains (e.g., political disinformation), so more development and testing will need to be done to bring these techniques into the securities landscape.
[86] ibid.
[87] ibid.
[88] The increase was isolated to the email scams, which the interactive prebunking intervention focused on, with no significant difference detected for the SMS and letter scams. The authors hypothesize that hallmarks of scams differ by channel and suggest the need for channel-specific interventions. Finally, when comparing effectiveness between immediate testing and 10 days after training, the prebunking condition was no longer statistically significant which indicates that the effect of this training decreases over time.
[89] Bullee, J., & Junger, M. (2020). How effective are social engineering interventions? A meta-analysis. Inf. Comput. Secur., 28, 801-830.
[90] While these findings are promising, the authors note that most of the studies evaluating the effectiveness of these games are proof of concepts or small-scale pilot studies and may not be sufficiently powered
[91] Twitter. (n.d.) Addressing misleading information.
[92] Saltz, E., Barari, S., Leibowicz, C. R., & Wardle, C. (2021). Misinformation interventions are common, divisive, and poorly understood. Harvard Kennedy School (HKS) Misinformation Review, 2(5).
[93] ibid.
[94] ibid.
[95] Pennycook, G., Bear, A., Collins, E. T., & Rand, D. G. (2020). The implied truth effect: Attaching warnings to a subset of fake news headlines increases perceived accuracy of headlines without warnings. Management Science, 66(11), 4944–4957.
[96] Epstein, Z., Foppiani, N., Hilgard, S., Sharma, S., Glassman, E.L., & Rand, D.G. (2021). Do explanations increase the effectiveness of AI-crowd generated fake news warnings? International Conference on Web and Social Media.
[97] ibid.
[98] Yaqub, W., Kakhidze, O., Brockman, M. L., Memon, N., & Patil, S. (2020). Effects of credibility indicators on social media news sharing intent. Proceedings of the 2020 CHI Conference on Human Factors in Computing Systems.
[99] ibid.
[100] Snijders, C., Conijn, R., de Fouw, E., & van Berlo, K. (2022). Humans and algorithms detecting fake news: Effects of individual and contextual confidence on trust in algorithmic advice. International Journal of Human–Computer Interaction, 39(7), 1483–1494.
[101] Lu, Z., Li, P., Wang, W., & Yin, M. (2022). The effects of AI-based credibility indicators on the detection and spread of misinformation under social influence. Proceedings of the ACM on Human-Computer Interaction, 6(CSCW2), 1–27. https://doi.org/10.1145/3555562
[102] Bitdefender. (n.d.) Bitdefender Scamio: The next-gen AI scam detector.
103] Bitdefender. (2023, December 14) Bitdefender Launches Scamio, a Powerful Scam Detection Service Driven by Artificial Intelligence
[104] Metadata is data that provides information about other data (e.g., the source of data)
[105] Kim, M., Song, C., Kim, H., Park, D., Kwon, Y., Namkung, E., Harris, I. G., & Carlsson, M. (2019). Scam detection assistant: Automated protection from scammers. 2019 First International Conference on Societal Automation (SA).
[106] Cross, C. (2016) Using financial intelligence to target online fraud victimisation: applying a tertiary prevention perspective. Criminal Justice Studies, 29(2), pp. 125-142.
Experimental Research
Experimental Research
To further our research, we conducted an experiment to examine 1) whether AI-enhanced scams are more harmful to retail investors than conventional scams, and 2) whether mitigation strategies can reduce the adverse effects of AI-enhanced scams by improving investors' ability to detect and avoid them.
We conducted a 4-arm randomized controlled trial (RCT) to examine whether the two different types of mitigation strategies can reduce susceptibility to investment scams.[107] The first mitigation strategy was an “inoculation”, while the second took the form of a web browser plug-in that labelled potentially fraudulent investment opportunities. The experiment also examined the extent to which AI-enhanced scams might attract more investment than conventional scams not enhanced by AI.
The sample comprised of 2,010 Canadian residents aged 18 or older. 58% of the sample were current investors[108] and 56% of participants completed the experiment on a mobile device. We confirmed groups were balanced across key demographic characteristics, such as gender (56% women & others) and age (median of 42 years). Additional demographic details are available in Appendix A.
In the experiment, research participants received $10,000 in simulated cash to invest across six opportunities presented on a simulated social media feed. Among the six opportunities, three social media posts promoted legitimate investment opportunities, while the other three promoted fraudulent investment opportunities.
The content and features of the social media feed depended on the experimental group to which participants were randomly assigned (see Table 1 for description, and Figures 7 to 10 for images of social media feed).
Condition | Description |
---|---|
Control 1 (C1): Conventional Scams | Participants viewed three posts promoting legitimate investment opportunities and three fraudulent posts which replicated conventional investment scams. The legitimate opportunities and the scams were based on posts identified in our environmental scan. They were representative of common approaches but all identifying details of the posts were disguised. |
Control 2 (C2): AI-enhanced Scams | Participants viewed the same 3 legitimate investment opportunities and 3 AI-enhanced versions of the conventional scam posts. To enhance the fraudulent opportunities, we used widely available low-to-no-cost AI tools to increase the sophistication of the scam. In one case, the AI-enhanced scam focused on the use of AI-driven trading algorithms (a key trend identified in the environmental scan). |
Treatment 1 (T1): Inoculation Mitigation | At the beginning of the experiment, participants saw a social media post from a trusted source (e.g., regulator) providing an example of a scam and listing common features of investment scams—the “inoculation” mitigation. Then, they viewed the same social media feed as Control 2 (3 legitimate opportunities and 3 AI-enhanced scams). |
Treatment 2 (T2): Web Browser Plug-in Mitigation | Participants viewed the same social media feed from Control 2. However, all the scam posts and one of the legitimate posts were labelled by a simulated web browser plug-in as being potentially fraudulent. Based on the number of common features of scams they exhibited, they were labelled as medium risk (in yellow) or high risk (in red). An estimated likelihood (%) of being a scam was also included. |
Table 1. The content and features of the social media feed for each participant group.
Legitimate Opportunities (All experiment groups)
Figure 7. The legitimate opportunities on the social media feed.
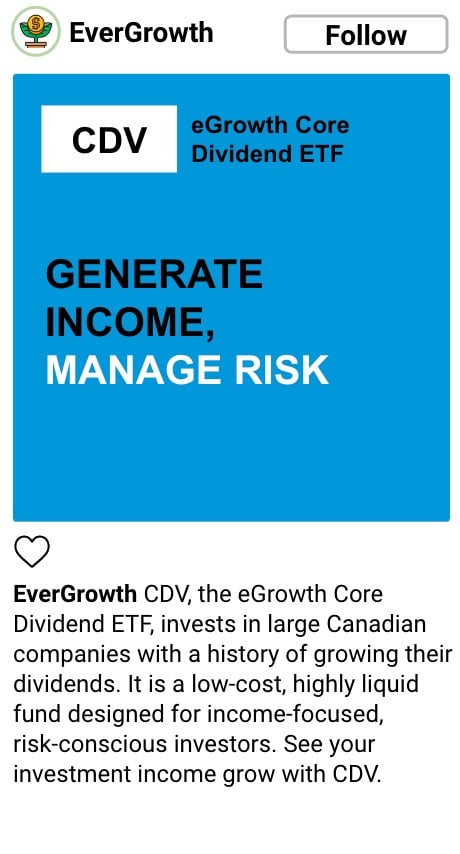
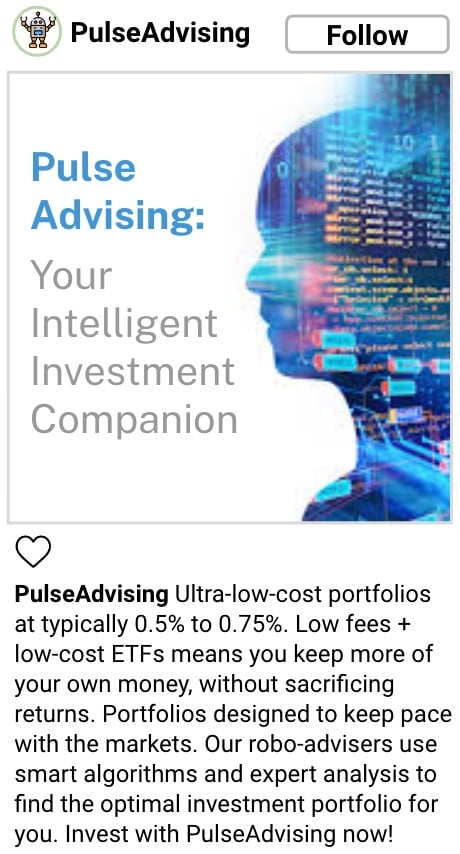
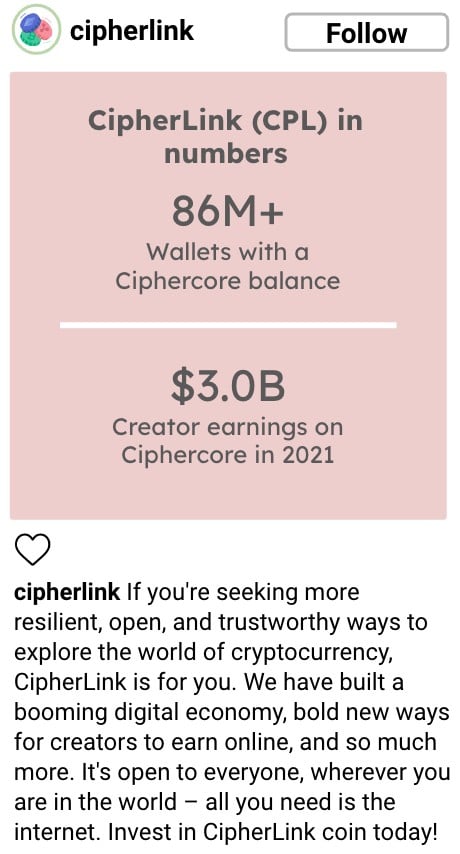
Conventional Fraudulent Opportunities (Only Control 1)
Figure 8. The conventional fraudulent opportunities on the social media feed.
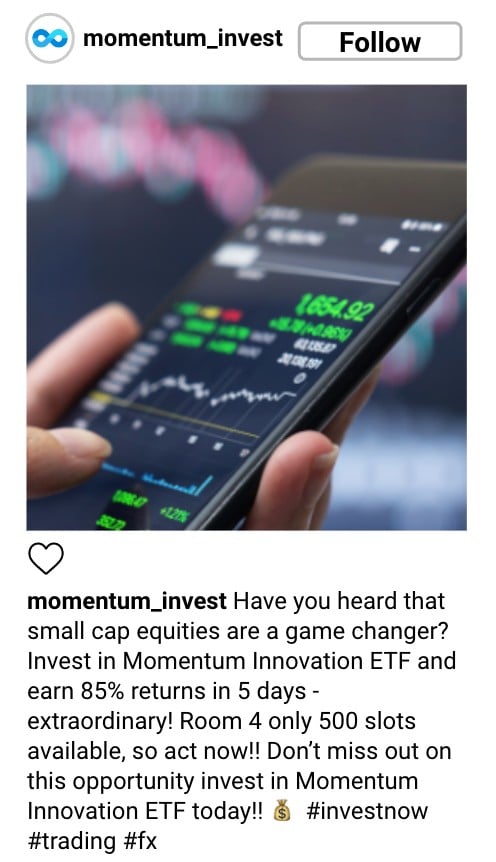
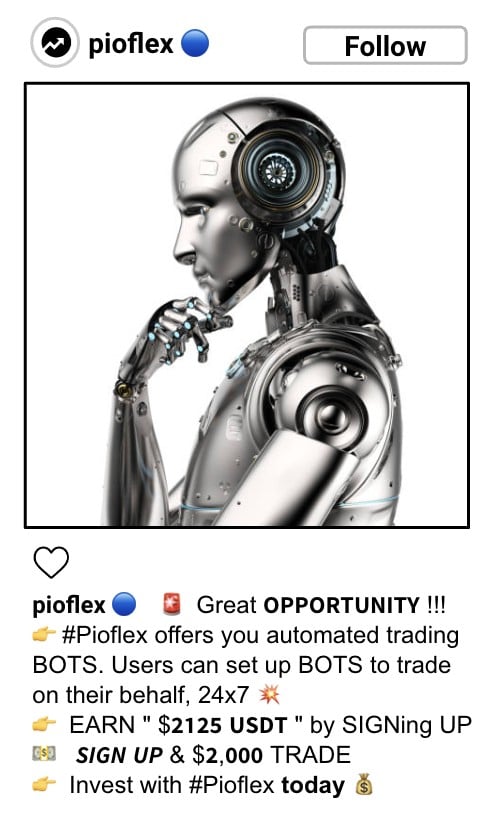
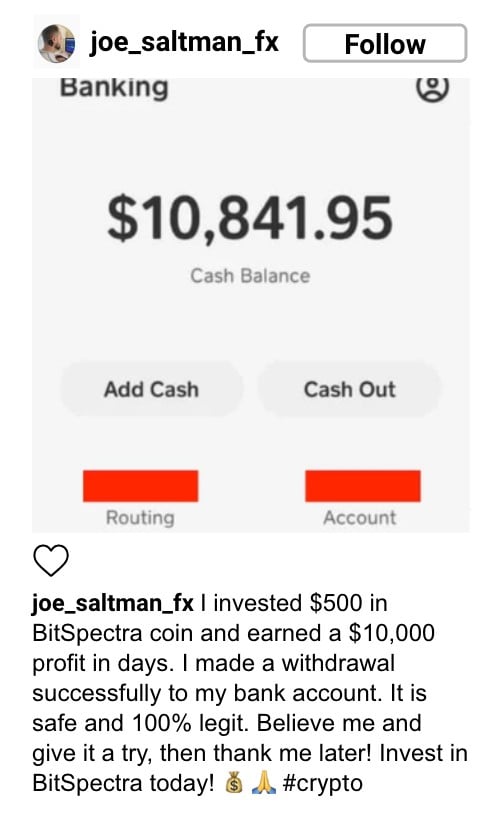
AI-enhanced Fraudulent Opportunities (Control 2; Treatments 1 and 2)
Figure 9. The AI-enhanced fraudulent opportunities on the social media feed.
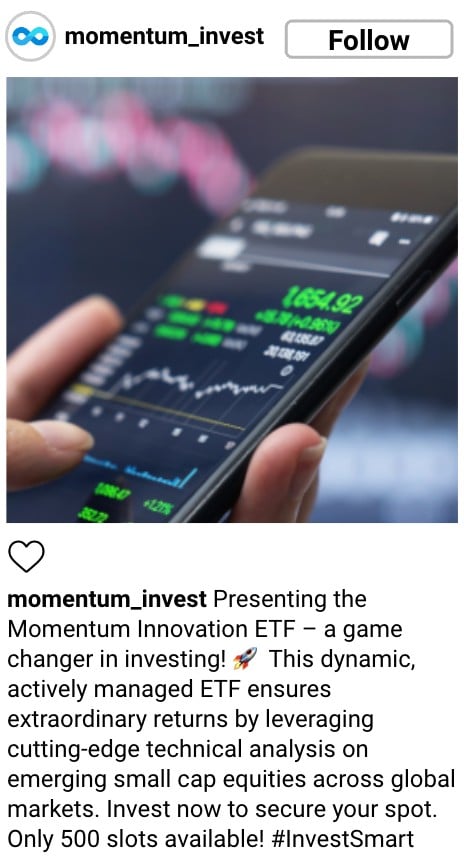
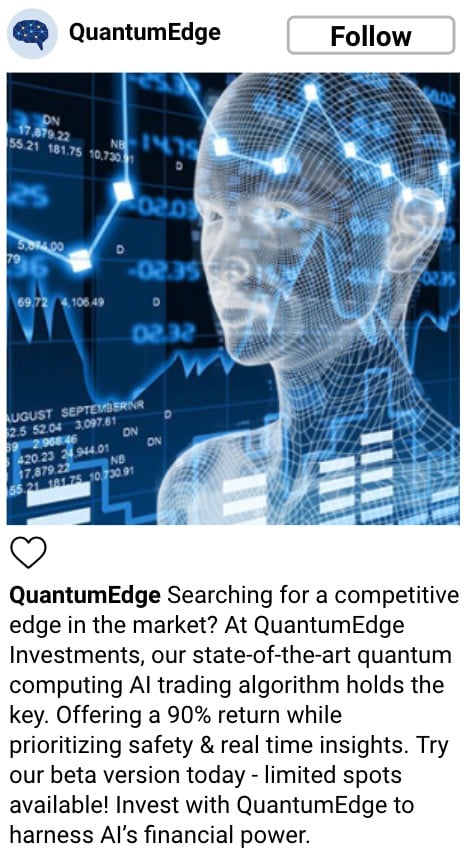
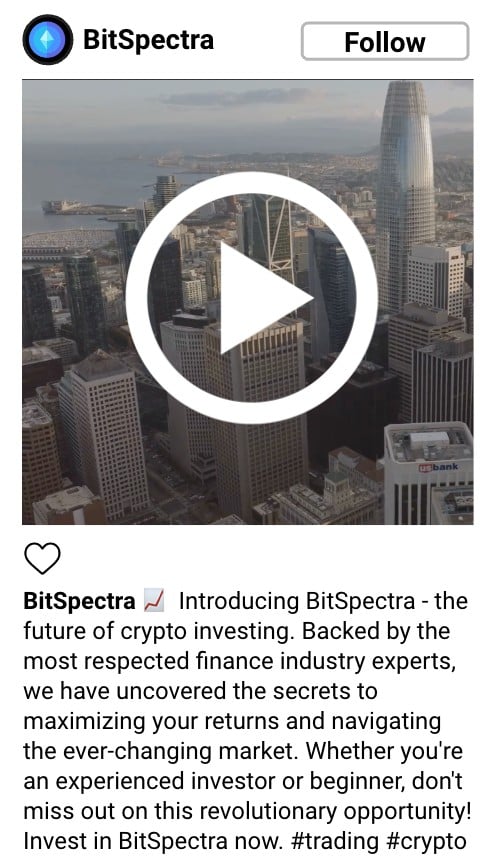
Mitigation Posts and Features (Treatments 1 and 2, respectively)
Figure 10. The mitigation posts on the social media feed.
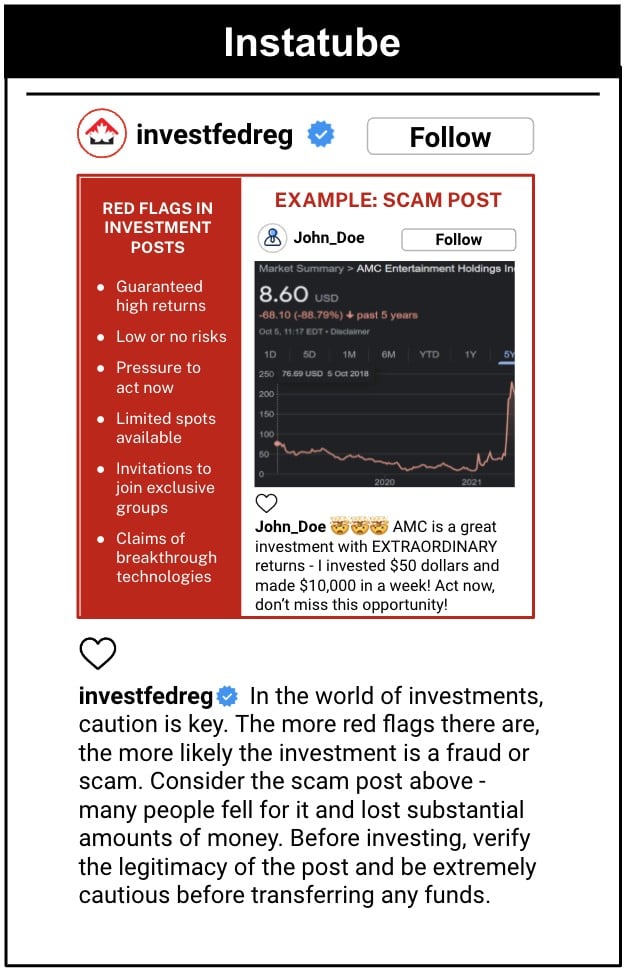

After participants viewed the opportunities in the social media feed, they were then instructed to invest their entire $10,000 across the opportunities presented, and to make this decision as if it were their own money and financial situation. To encourage more thoughtful and realistic allocations, participants were compensated partially based on the performance of their investments after 12 simulated months (and informed of this prior to completing the task). Once participants completed the investing activity, they received feedback on their investment performance based on their selection and were notified that some opportunities were fraudulent. The median completion time for the experiment was 5.50 minutes (mean= 8.24 minutes). See Appendix B for screenshots of each experimental.
After data collection, we analyzed how the amount invested in fraudulent opportunities differed between:
- Participants exposed to conventional scams compared to those exposed to AI-enhanced scams and,
- Participants exposed to AI-enhanced scams compared to those exposed to the same scams and a mitigation strategy.
We used an ordinary least squares (OLS)[109] regression to assess the impact of treatment assignment on the amount invested in fraudulent investment opportunities, controlling for age, gender, investment knowledge, and investor status.
[107] Randomized controlled trials (RCTs), widely regarded as the “gold standard” in scientific research, are rigorous experimental designs that randomly assign participants to treatment and control groups, ensuring unbiased comparisons. RCTs are valued for their ability to establish causality.
[108] Investors were defined as such by holding at least one of: individually held stocks, ETFs, securities, or derivatives, bonds, or notes other than Canada Savings Bonds, mutual funds, or private equity investments.
[109] Ordinary Least Squares (OLS) is a method used in statistics to find the best fit line for a set of data points. It tries to draw a line through a scatter plot of points in a way that keeps the line as close as possible to all the points.
Primary Results: Amount invested in fraudulent opportunities
Our primary outcome of interest was the total amount of money invested in the 3 fraudulent investment opportunities (of the 6 total opportunities). We analyzed whether investors would be more susceptible to AI-enhanced scams and to what extent two mitigation techniques (an inoculation and a web-browser plug-in) would reduce susceptibility to the AI-enhanced scams. Our key findings include:
- Participants invested 9 percentage points (pp)[110] more in AI-enhanced scams than in conventional scams (2% increase).
- Both mitigation strategies we tested were effective at reducing susceptibility to AI-enhanced scams.
- The “inoculation” strategy reduced the amount of money invested by 5pp (10% decrease),
- The web-browser plug-in reduced investments by 17pp (31% decrease).
AI-enhanced investment scams are more effective than conventional scams
As shown in Figure 11, participants exposed to AI-enhanced scams invested significantly more in fraudulent opportunities[111] than those exposed to conventional scams, illustrating the significant risk that generative AI tools—when used with malintent—may pose to investors. This finding suggests that by using widely available generative AI systems to enhance their materials, scammers can effectively ‘turbocharge’ their scams to make them appear more attractive to retail investors. In particular, scammers can enhance original scam materials by enhancing the persuasive appeal of language, generating more compelling media, and highlighting the promise of AI.
While the data generated by our experiment are compelling, they may underestimate the full effect of AI systems in the real world. As generative AI applications become more powerful, available, and lower cost, scams will become more compelling, scalable, complex, and harder for investors to detect. Furthermore, we were only able to test a subset of the techniques available to scammers. “Deepfakes” and other cloning technology, which would have been inappropriate for us to test, could pose an even greater risk and can now be developed and deployed in minutes.
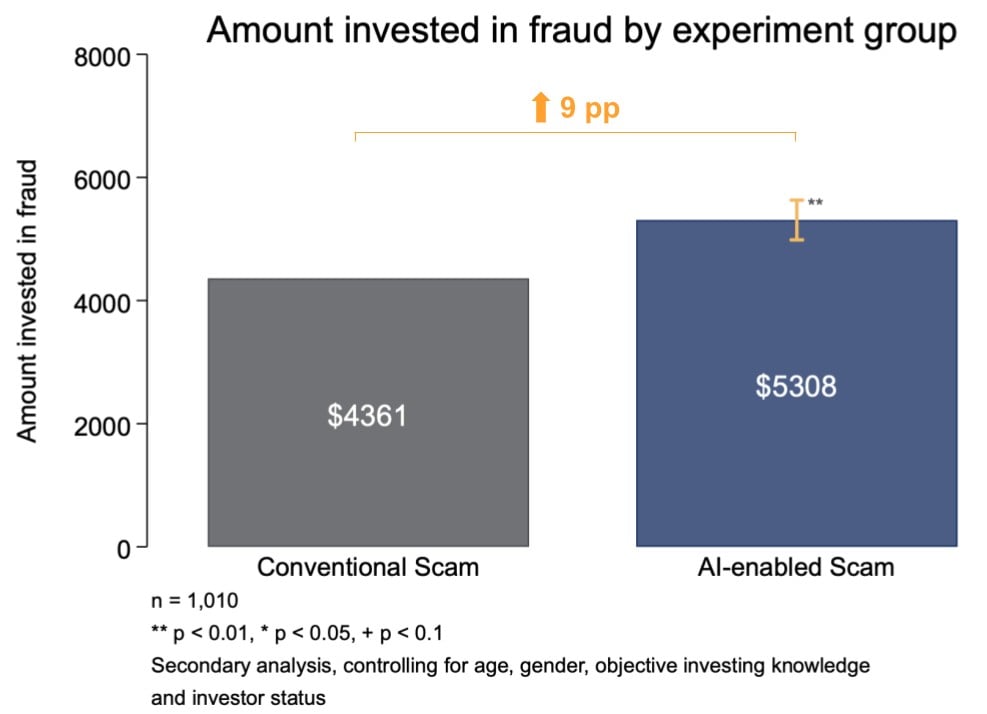
Both the inoculation and web-browser mitigations significantly reduced the amount invested in AI-enhanced scams.
The inoculation mitigation reduced the amount invested by 5pp (10% decrease), a moderate statistical effect, and the web browser mitigation decreased the amount invested by 17pp (31% decrease), a large statistical effect (See Figure 12). These differences were both statistically significant, as was the difference between the inoculation and web browser strategies.
As both inoculation and labelling mitigations[112] have been tested primarily against political misinformation, we show strong evidence that these mitigations are also effective in the securities context. By highlighting the hallmarks of investment scam—either just before or when people are exposed to them—we can reduce investor susceptibility to compelling investment scams.
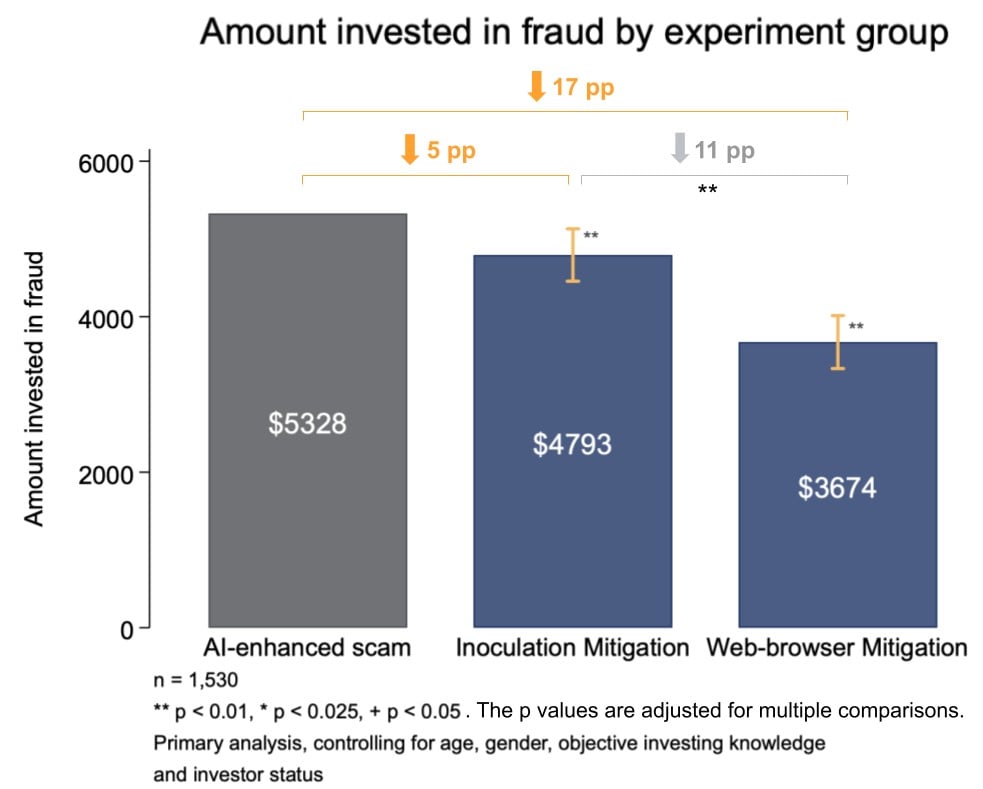
The results for the inoculation mitigation show that relevant, clear educational materials provided before people review investment opportunities can reduce the magnitude of harm posed by (AI-enhanced) investment scams. These materials should help investors identify key features of investment scams. Ideally, they should be readily available and accessible when making investment choices, regularly updated to reflect new trends or tactics, and clearly linked to a trusted and authoritative source. This inoculation technique could be applied in different ways, for instance it could be implemented as an advertisement within social media platforms, such as Instagram or X.
While an effective technique, inoculation strategies have some limitations. First, the effect sizes—the magnitude of differences between experiment groups—are moderate, both in our own experiment and the broader literature. Second, the broader literature suggests that the impact of this technique decays over time. Third, the impact of the inoculation will depend on how closely the content and channel of the message match the context of the scam. Fourth, for inoculations to be effective, investors need to see or access the inoculation, understand the information being shared, and remember that information when presented with a scam. Given the relatively low cost of disseminating inoculation content, the use of this strategy is still recommended—despite of its limitations.
A web browser extension or plug-in that labels potential scams in situ would address many of the limitations specific to inoculations. Our experiment suggests that impact for a web browser plug-in could be quite strong. This type of solution reduces barriers related to accessing the educational material and remembering it in the moment that investors are presented with opportunities. It can offer highly salient, context-specific, and repeated visual cues. Our environmental scan indicated that such solutions are not currently on the market but are capable of being developed. As a proof point, Bitdefender, a cybersecurity firm, has developed an AI-generated chatbot that can be used to detect online scams. By inputting text, emails, images, links, or even QR codes into the tool, the chatbot can analyze and flag potential threats to users. The most powerful mechanism for adoption at scale would be to include the plug-in by default in browsers and/or social media apps.
Beyond labelling potential scams in situ, we also demonstrate that the timely warnings of scams and the messaging within those warnings could be effective at mitigating scams. This type of messaging could be used within education materials and within advertisements in response to certain search results. For example, these warnings could appear as Google search ads when users search for investments that have already been identified as scams.
Exploratory Results
Likelihood of investing in one or more fraudulent opportunities
The mitigations we tested not only reduced the amount people invested in the fraudulent opportunities but also the likelihood of investing in any of them. As shown in Figure 13, both inoculation and web-browser mitigations reduced the proportion of individuals who invested in at least one AI-enhanced scam. The inoculation mitigation reduced this likelihood by 4% while the web-browser mitigation reduced of the likelihood by 18%.
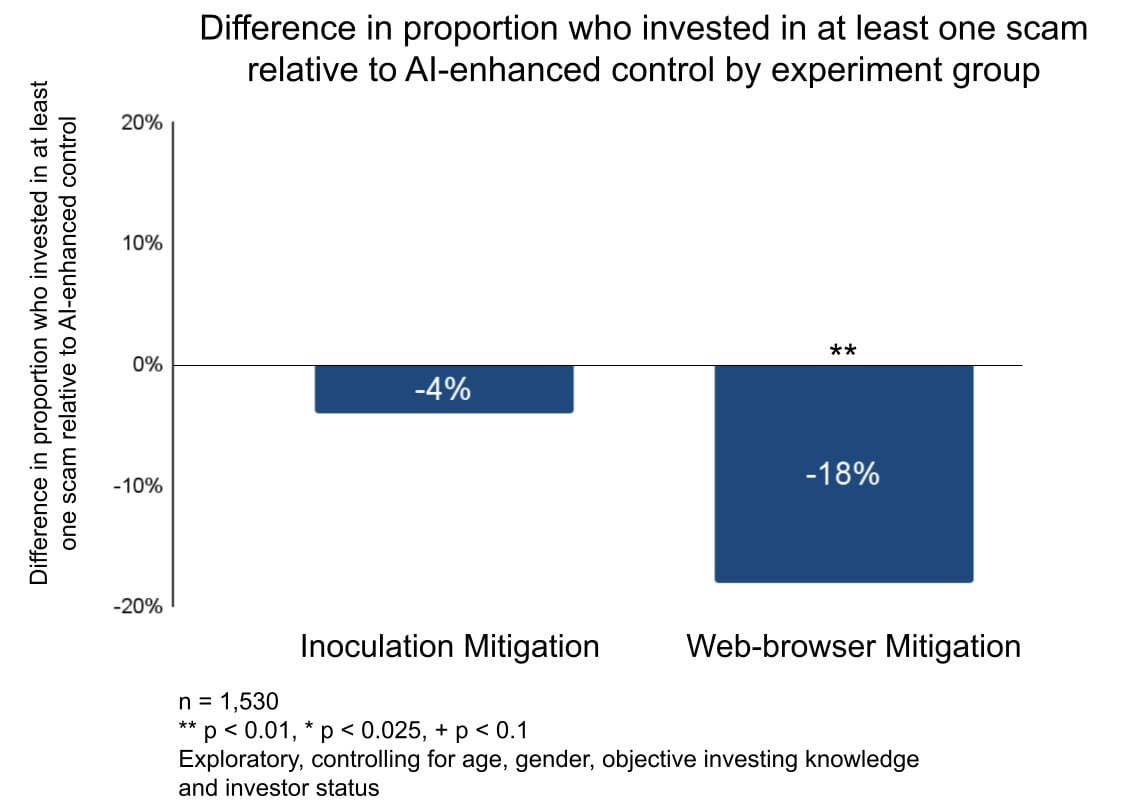
Effect of different risk-level labels
In the web-browser mitigation, investment opportunities had either a “red” label, signifying a high probability of being fraudulent, or a “yellow” label, signifying a moderate probability of being fraudulent, or no label at all. Table 2 shows a descriptive analysis suggesting that the colour of the label did not have a material effect on the amount invested in scams (red = $2405.69; yellow = $2333.06). While not definitive, this indicates that the presence of a visual cue, not its content, is most important. The lack of nuance in investor reaction to labels, if supported by further research, has significant implications. For example, in our experiment, one of the legitimate investment opportunities received a yellow label, as it contained one hallmark of a scam. If legitimate opportunities are inaccurately labelled, even as moderate risk, we anticipate a significant investor reaction and corresponding deterrence. Further research is needed to confirm these hypotheses as the posts with “red” and “yellow” labels have a relatively higher standard deviation than those without a label, indicating that there is more variability in how much participants invested in these labelled opportunities.
Amount allocated to opportunities for each label
Label | Mean | Standard Deviation |
---|---|---|
Red Label | 2405.69 | 2556.48 |
Yellow Label | 2333.08 | 2589.67 |
No Label | 5261.23 | 3375.02 |
N = 489
Table 2. Amount allocated to each risk-level label in the web-browser mitigation condition.
Demographic considerations
Our data set from this experiment included variables related to the demographic characteristics of the participants. While sample sizes were not large enough to conduct detailed analyses of these subgroups, our descriptive exploration of the data suggests a few interesting avenues for further research:
- Men appear to be more susceptible to scams; they allocated $4709 to investment scams compared to $4431 among women and other participants.
- Individuals with greater financial knowledge appear less susceptible to scams; those who scored 3/3 on a set of objective knowledge questions allocated $4239 to investment scams, while those who correctly answered 0/3 or 1/3 questions allocated $4875 and $4921, respectively.
These findings are in line with statistics showing that in Canada, young men and investors with limited financial literacy are more susceptible to scams.[114]
- While the inoculation and browser plug-in mitigations were generally effective across groups, they appear more effective for women and others. Women and other respondents reduced the amount allocated to the fraudulent opportunities by $1905 in the web-browser mitigation, while this was only $1345 among men.
This data and other descriptive information about subgroups can be found in Appendix A.
[111] Percentage points are a unit of change in a percentage. If a rate increases from 10% to 15%, that’s a rise of 5 percentage points.
[112] hat helps us determine if the results of an experiment are statistically significant. If the p-value is small (typically under 0.05), it means that if we were to repeat this experiment under the same conditions, we will likely find the same results again.
[113] The web browser plug-in we simulated represents a form of labelling mitigation.
[114] The AI-enhanced scam group value is slightly different for Figures 11 and 12. In Figure 11, the AI-enhanced scam is the “treatment” group, which requires an adjusted value, whereas in Figure 12, the AI-enhanced scam is the control, which has an unadjusted value. The adjusted treatment values account for other variables (covariates) in the regression model to reflect the impact of the treatment more precisely.
The experiment was conducted using an online platform that simulated real-world investment opportunities and decisions. While the design of the experiment used a variety of tools to enhance the generalizability of the results to the real world (e.g., variable incentives, attention checks, replication of real-world investment content, etc.), there are some limitations that should be considered when interpreting the results:
- Cues of legitimacy and scams. In real-world settings there may be additional cues to help people differentiate legitimate and fraudulent opportunities (e.g., incorrect links or comments on the social media post).
- Requirement to invest. Participants in the experiment were required to invest the full amount they were allocated. In a natural investing context, investors are not required to invest in any opportunity.
However, we hypothesize that these limitations do not directly affect our primary research question, which was: how the mitigation strategies influence investment choices (and the extent to which AI enhances scams). The most significant limitation of our experiment is that participants had much less at stake in selecting investment opportunities, as there is no real loss in our experiment—just minor incentives for better performance. In the real world, the potential losses are real and far greater, and so the impact of AI-enhanced scams could be much greater.
Conclusion
Conclusion
The use of AI in the retail investing space is rapidly expanding. While AI as a technology is neither inherently good nor bad from an investor protection perspective, the use of AI could bring new threats to investor welfare when applied to scams. Malicious actors are exploiting the advanced capabilities of AI to manipulate markets, deceive investors, and orchestrate fraudulent schemes—posing significant risks to the integrity of financial markets. This concern is further amplified when considering the findings from our previous report on Artificial Intelligence and Retail Investing[115], which noted that retail investors adhered to advice from AI advisors similarly to human advisors. If retail investors trust AI advice as much as they do human advice, then poor, misleading, and/or manipulative AI advice could present substantial retail investor protection concerns.
The current research report was designed to assess the current level of risk associated with AI-enabled scams, and determine a responsive, evidence-based path forward for investor protection. Our research was conducted in two phases. First, we conducted desk research, which revealed they various ways AI capabilities could be exploited by malicious actors to more effectively deceive investors. Generative AI technologies are “turbocharging” common investment scams by increasing their reach, efficiency, and effectiveness. New scams are also being developed that were impossible without AI (e.g., deepfakes and voice cloning) or that exploit thepromise of AI through false claims of ‘AI-enhanced’ investment opportunities. Together, these enhanced and new types of investment scams are creating an investment landscape where they are more pervasive, harder to detect, and potentially more damaging.
We also explored evidence-based strategies to mitigate the harms associated with AI-enhanced or AI-related investment scams. We explored two sets of mitigations: system-level mitigations, which are designed to limit the risk of scams across all (or a large pool of) investors, and individual-level mitigations, which are designed to empower or support individual investors in detecting and avoiding scams. Drawing from research in various online contexts, including targeting misinformation/disinformation, we identified specific measures tailored for AI-enhanced scams, as well as broader strategies applicable to this domain and others.
In the second phase of our research, we built an online investment simulation to empirically test investors’ susceptibility to fraudulent investment opportunities and the effectiveness of mitigation strategies designed to protect investors from these harms. The experiment generated critical, novel, and policy-relevant insights:
- AI-enhanced scams pose significantly more risk to investors compared to conventional scams. Participants invested 22% more in AI-enhanced scams than in conventional scams. This finding suggests that using widely available generative AI tools to enhance materials can make scams much more compelling. These findings reinforce the critical and escalating threat posed to investors by the availability of generative AI tools in executing scams.
Mitigations can reduce the magnitude of harm posed by AI-enhanced scams. In particular, a web browser plug-in that flags potential scams could quite effective. Both mitigation strategies we tested were effective at reducing susceptibility to AI-enabled scams. The ‘“inoculation” strategy reduced the amount invested in fraudulent opportunities by 5pp (10% decrease) while the web-browser plug-in reduced investments by 17pp (31% decrease).
These results suggest that relevant, clear educational materials provided before people review investment opportunities can reduce the magnitude of harm posed by (AI-enhanced) investment scams. This inoculation technique could be implemented as an advertisement within social media platforms, such as Instagram or X.
We also present significant empirical and theoretical support for the development of a browser or app-based, AI-driven scam detection tool. Beyond labelling potential scams in situ, this type of messaging could be used within education materials and within advertisements in response to certain search results. For example, these types of warnings could appear as Google search ads when users search for investments that have already been identified as scams.
[115] Ontario Securities Commission (2024), Artificial Intelligence and Retail Investing: Use Cases and Experimental Research.
Authors & Appendices
Authors
Ontario Securities Commission:
Matthew Kan
Senior Advisor, Behavioural Insights
[email protected]
Patrick Di Fonzo
Senior Advisor, Behavioural Insights
[email protected]
Meera Paleja
Program Head, Behavioural Insights
[email protected]
Kevin Fine
Senior Vice President, Thought Leadership
[email protected]
Behavioural Insights Team (BIT):
Amna Raza
Senior Advisor
[email protected]
Riona Carriaga
Associate Advisor
[email protected]
Sasha Tregebov
Director
[email protected]
All values in this appendix are unadjusted, descriptive means.
Primary Analysis: Amount allocated to fraudulent opportunities
Amount allocated to fraudulent opportunities (unadjusted)
Group | Mean | Standard Deviation | Observations |
---|---|---|---|
Conventional Scam | 4360.66 | 2794.39 | 480 |
AI-enhanced Scam | 5327.86 | 2460.77 | 530 |
Inoculation Mitigation | 4770.73 | 2764.87 | 511 |
Web-Browser Mitigation | 3681.55 | 3099.90 | 489 |
N = 2,010
Average amount allocated to fraudulent opportunities by treatment group and demographic group
Conventional Scam | AI-enhanced Scam | Inoculation Mitigation | Web-Browser Mitigation | Total | |
---|---|---|---|---|---|
Total | 4360.66 | 5327.86 | 4770.73 | 3681.55 | 4554.73 |
Gender | |||||
Men | 4400.09 | 5389.24 | 4977.76 | 4043.97 | 4708.61 |
Women and other | 4327.58 | 5280.44 | 4614.21 | 3375.20 | 4431.46 |
Age | |||||
18-24 | 4778.36 | 5661.27 | 4878.90 | 3490.14 | 4719.79 |
25-44 | 3932.54 | 5248.21 | 5097.48 | 3528.17 | 4482.66 |
45-64 | 4865.64 | 5343.48 | 4486.02 | 4114.37 | 4709.33 |
65+ | 3865.28 | 5179.23 | 4353.51 | 3262.5 | 4237.66 |
Investor Status | |||||
Non-investor | 4628.86 | 5208.13 | 4621.51 | 3560.46 | 4527.52 |
Investor | 4155.57 | 5410.223 | 4881.75 | 3761.87 | 4574.14 |
Financial Knowledge | |||||
0 / 3 questions answered correctly | 5103.90 | 5788.74 | 4612.95 | 3887.5 | 4874.92 |
1 / 3 questions answered correctly | 5106.38 | 5588.86 | 4828.9474 | 4128.68 | 4921.47 |
2 / 3 questions answered correctly | 4150.71 | 5091.23 | 4884.15 | 3796.91 | 4508.98 |
3 / 3 questions answered correctly | 3930.34 | 5378.96 | 4588.65 | 3090.12 | 4239.07 |
Final portfolio value after 12 simulated months by condition
Group | Mean | Standard Deviation | Observations |
---|---|---|---|
Conventional Scam | 6485.27 | 3213.53 | 480 |
AI-enhanced Scam | 5372.97 | 2829.89 | 530 |
Inoculation Mitigation | 6013.68 | 3179.60 | 511 |
Web-Browser Mitigation | 7266.25 | 3564.89 | 489 |
N = 2,010
Amount allocated to fraudulent opportunities for T2 (Web-browser Mitigation)
Label | Mean | Standard Deviation | Observations |
---|---|---|---|
Red Label | 2405.69 | 2556.48 | 489 |
Yellow Label | 2333.08 | 2589.67 | 489 |
No Label | 5261.23 | 3375.02 | 489 |
N = 489
Background Questions and Demographics
Gender
Men | 44.48% |
---|---|
Women and other | 55.52% |
N = 2,010
Age
18-24 | 14.98% |
---|---|
25-44 | 39.70% |
45-64 | 31.29% |
65+ | 14.03% |
N = 2,010
Investor Status
Investor | 58.36% |
---|---|
Not an investor | 41.64% |
N = 2,010
Financial Knowledge Score
0/3 questions correctly answered | 8.16% |
---|---|
1/3 questions correctly answered | 29.00% |
2/3 questions correctly answered | 45.02% |
3/3 questions correctly answered | 25.97% |
N = 2,010
Highest Level of Education Completed
High school graduate or less | 23.63% |
---|---|
Some college or training | 34.28% |
Bachelor’s degree and above | 42.09% |
N = 2,010
Platform used to complete experiment | |
Mobile phone | 56.00% |
Desktop computer | 44.00% |
N = 2,010
Household Annual Income Before Taxes
Less than $34,999 | 26.87% |
---|---|
$35,000 to $54,999 | 20.10% |
$55,000 to $59,999 | 5.17% |
$60,000 to $79,999 | 13.83% |
$80,000 to $99,999 | 11.39% |
$100,000 to $124,999 | 10.00% |
$125,000 to $149,999 | 12.64% |
N = 2,010
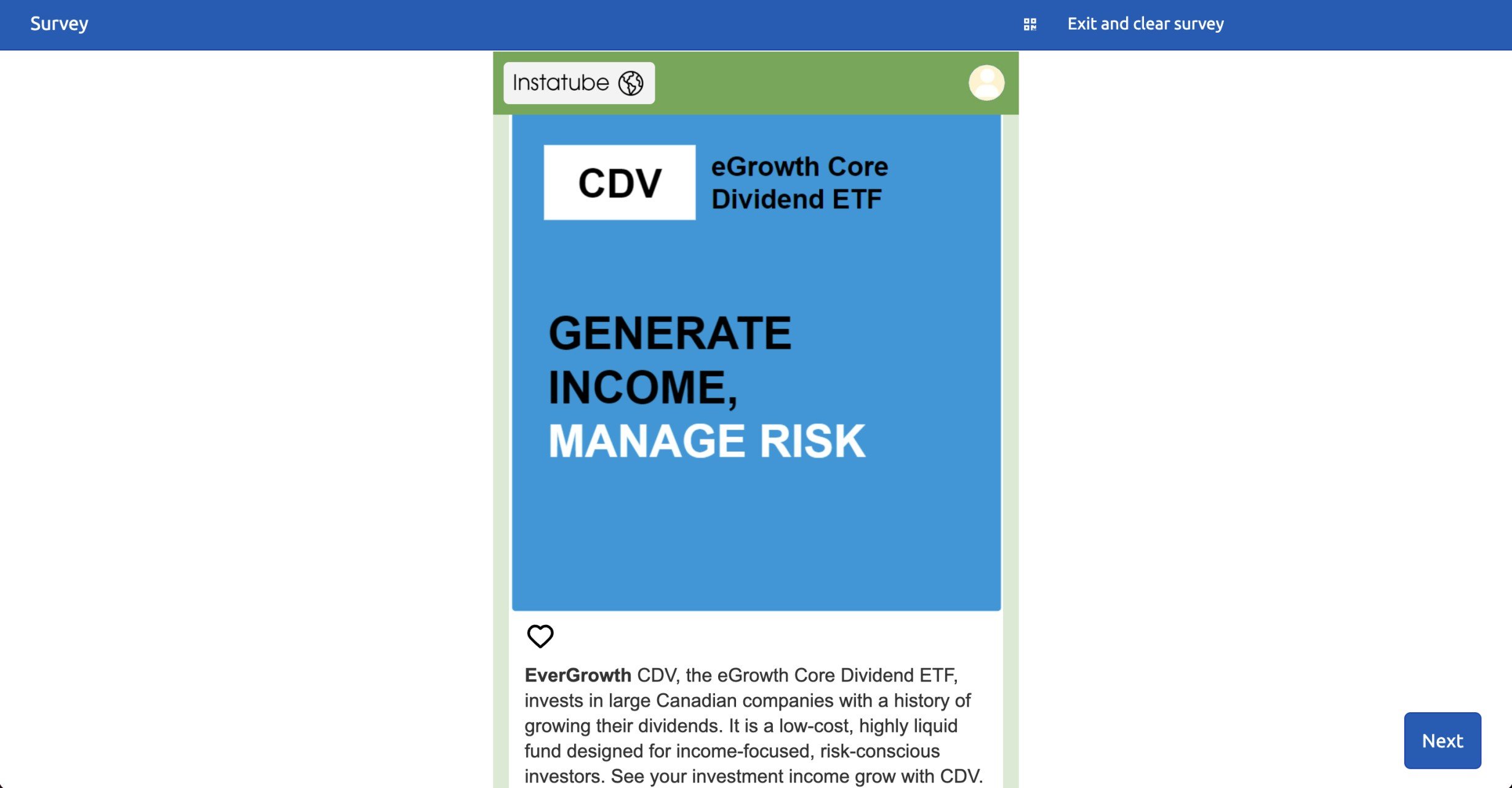
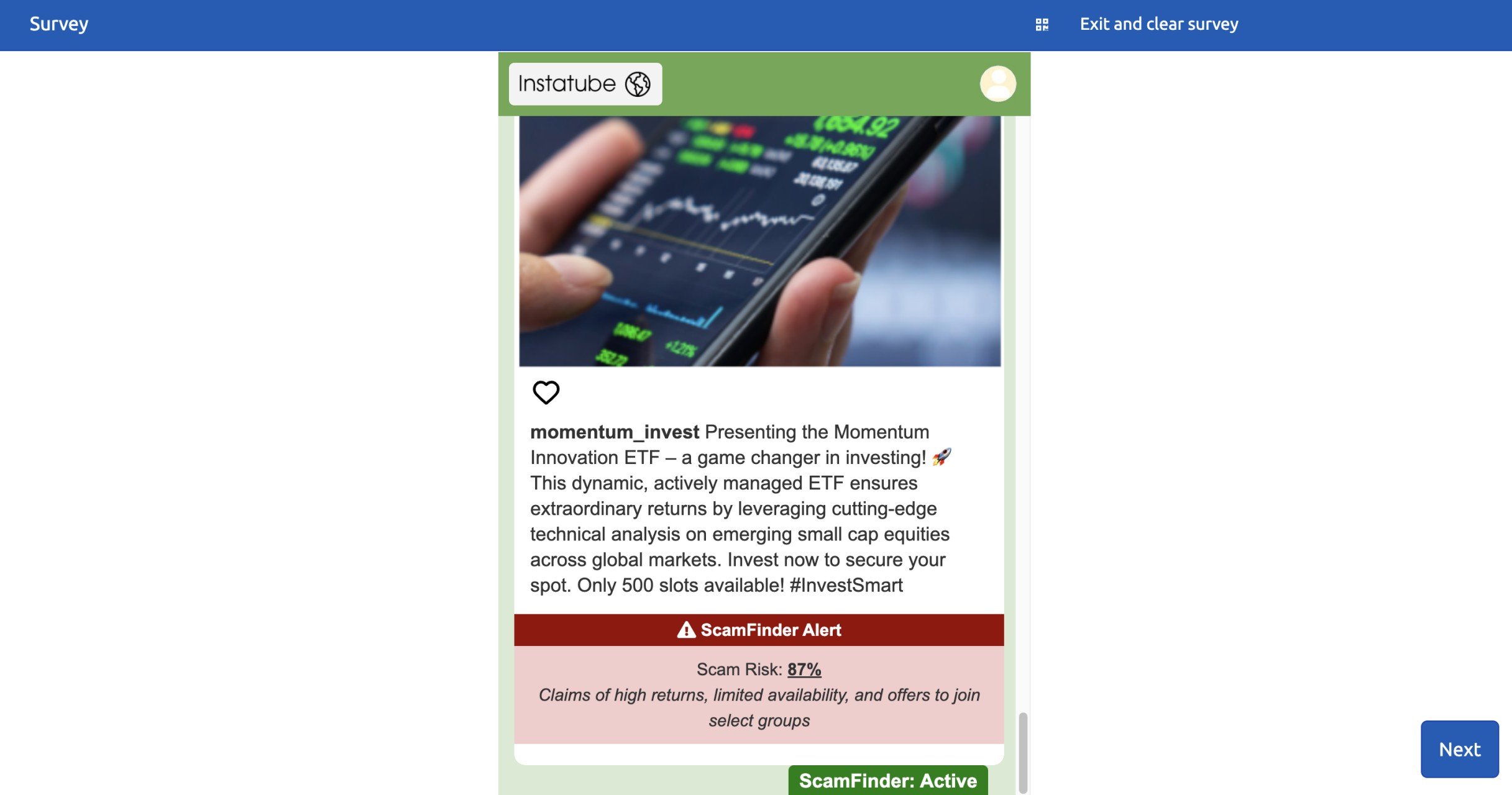
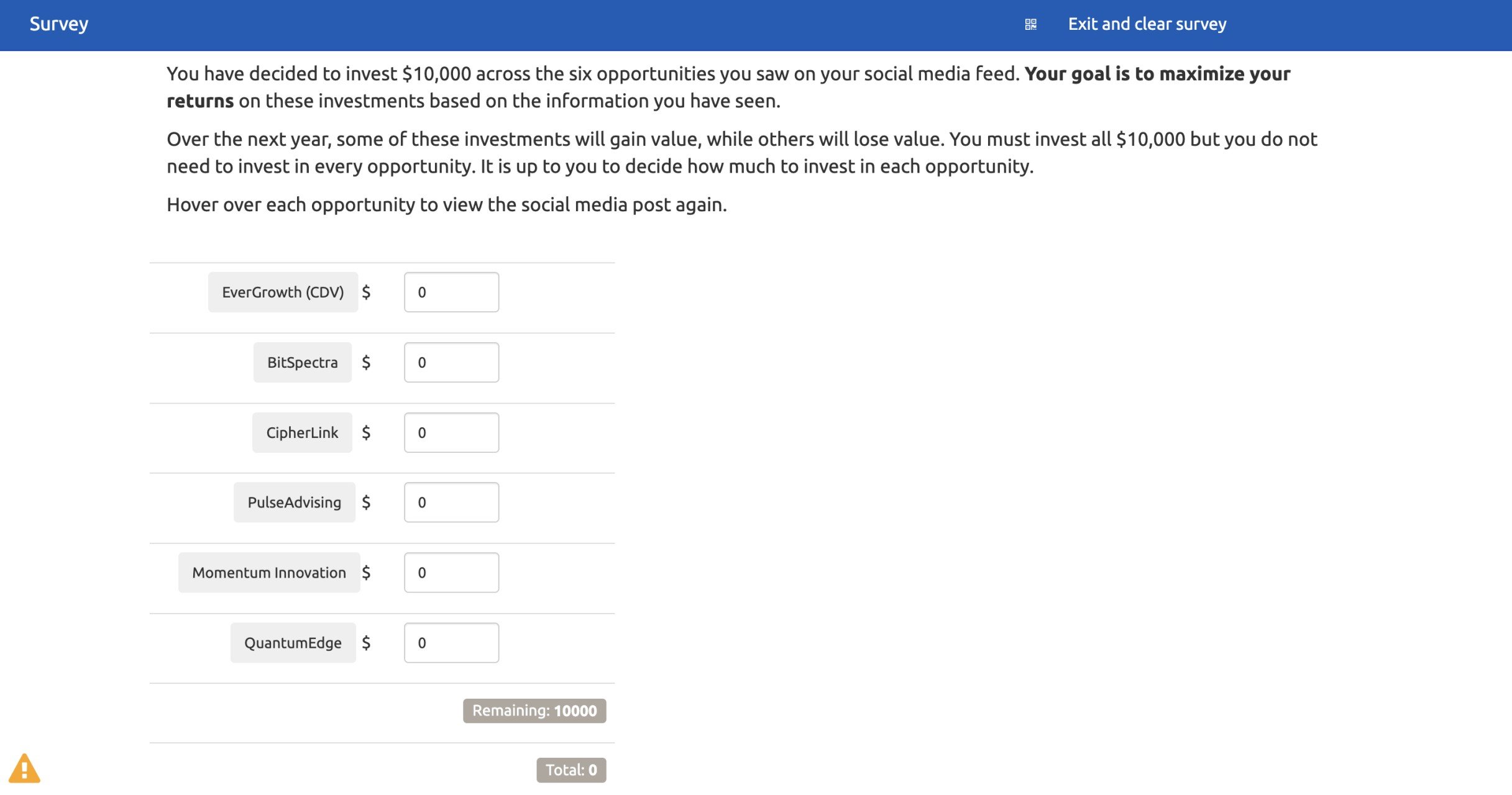
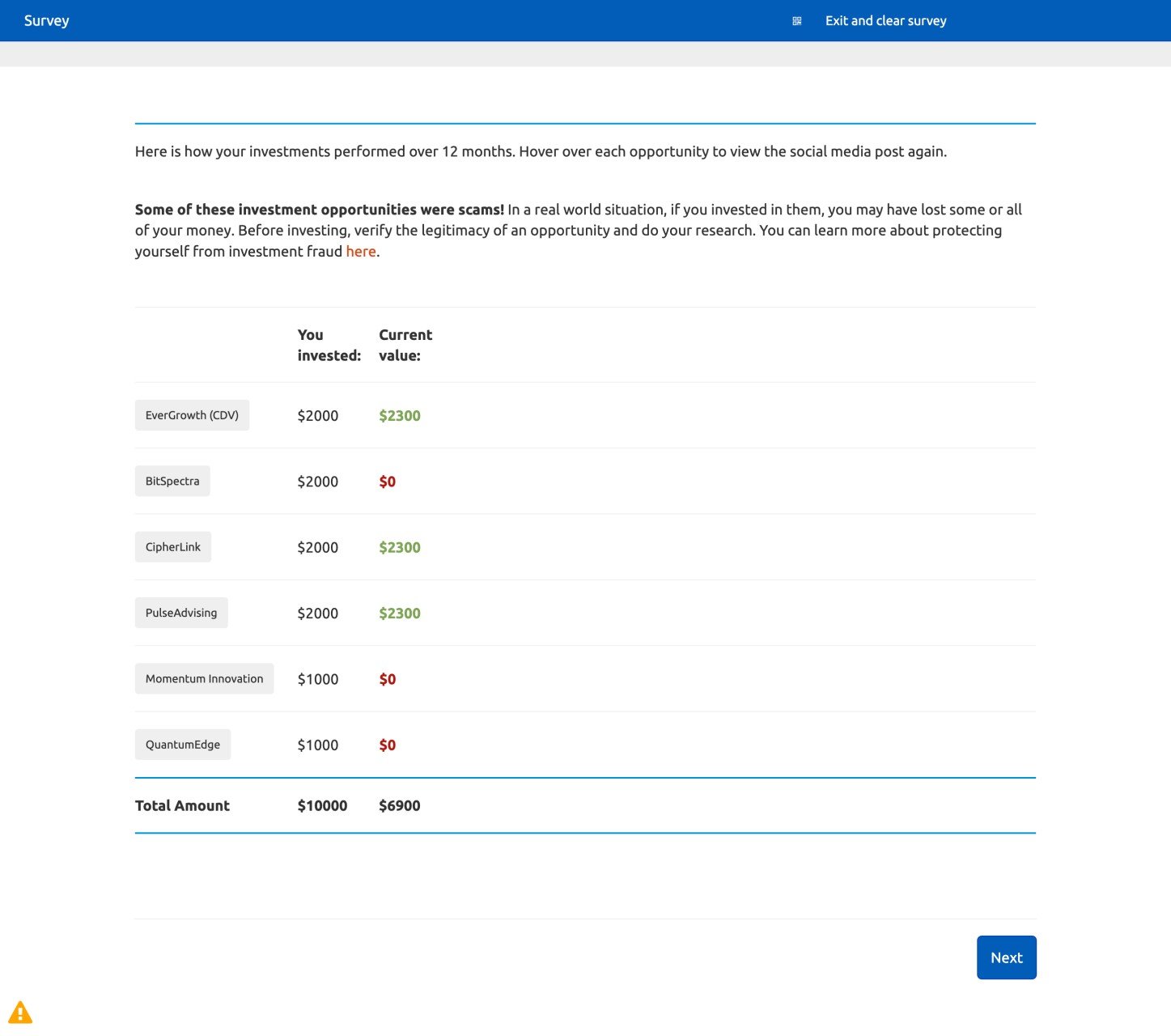
Anderljung, M. and Hazell, J. (2023, March 16). Protecting Society from AI Misuse: When are Restrictions on Capabilities Warranted? Centre for the Governance of AI.
APWG (2020). APWG Phishing Attack Trends Reports.
Asia News Network. (2023, September 6). Rise of AI-based scams.
Bateman, J. (2020, July). Deepfakes and Synthetic Media in the Financial System: Assessing Threat Scenarios. Carnegie Endowment for International Peace.
Bitdefender. (2023, December 14) Bitdefender Launches Scamio, a Powerful Scam Detection Service Driven by Artificial Intelligence
Bitdefender. (n.d.) Bitdefender Scamio: The next-gen AI scam detector.
Bontridder, N., & Poullet, Y. (2021). The role of Artificial Intelligence in disinformation. Data & Policy, 3. https://doi.org/10.1017/dap.2021.20
Brewster, Thomas. (2021). Fraudsters Cloned Company Director’s Voice In $35 Million Heist, Police Find. Forbes.
British Columbia Securities Commission. (2022). Evolving Investors: Emerging Adults and Investing.
Brundage, M., et al. (2018). The malicious use of artificial intelligence: Forecasting, prevention, and mitigation. Preprint arXiv:1802.07228, 2018.
Buchanan, B., et al. (2021). Truth, lies, and automation: How language models could change disinformation. Center for Security and Emerging Technology.
Bullee, J., & Junger, M. (2020). How effective are social engineering interventions? A meta-analysis. Inf. Comput. Secur., 28, 801-830.
Burke, J. & Kieffer, C. (2021). Can Educational Interventions Reduce Susceptibility to Financial Fraud?. FINRA Investor Education Foundation.
Carleson, C. (2023, June 12). First Annual Study: The 2023 State of Investment Fraud. Carlson Law.
Chang, E. (2023, March 24). Fraudster’s New Trick Uses AI Voice Cloning to Scam People. The Street.
Choudhary, A. (2023, June 23). AI: The Next Frontier for Fraudsters. ACFE Insights.
Cross, C. (2016) Using financial intelligence to target online fraud victimisation: applying a tertiary prevention perspective. Criminal Justice Studies, 29(2), pp. 125-142.
Damiani, J. (2019). A Voice Deepfake Was Used To Scam A CEO Out Of $243,000. Forbes.
Day Pitney LLP. (2023, December 11). Estate Planning Update Winter 2023/2024 - The Good, the Bad, and the…Artificial? AI-enabled Scams: Beware and be Prepared. Day Pitney Estate Planning Update.
Drenik, G. (2023, October 11). Generative AI is Democratizing Fraud. What Can Companies And Their Consumers Do To Prevent Being Scammed? Forbes.
Epstein, Z., Foppiani, N., Hilgard, S., Sharma, S., Glassman, E.L., & Rand, D.G. (2021). Do explanations increase the effectiveness of AI-crowd generated fake news warnings? International Conference on Web and Social Media.
Ferrer et al. (2020, June 12). Deepfake Detection Challenge Results: An open initiative to advance AI. Meta.
Fletcher, E. (2023, October 6). Social media: a golden goose for scammers. Federal Trade Commission.
Foran, Pat. (2022). This is how an Ontario woman lost $750,000 in an Elon Musk deep fake scam. CTV News.
Foran, Pat. (2023). 'Trudeau said that he invested in the same thing:' How a deepfake video cost an Ontario man $11K US. CTV News.
Global Times. (2023, June 26). China’s legislature to enhance law enforcement against ‘deepfake’ scam. Global Times.
Goldstein, J.A., et. al. (2023, January). Generative Language Models and Automated Influence Operations: Emerging Threats and Potential Mitigations. Georgetown Center for Security and Emerging Technology.
Government of Canada. (2023). Investment Scams: What’s in a fraudster’s toolbox?.
Harrar, S. (2022, March 9). Crooks Use Fear of Missing Out to Scam Consumers. American Association of Retired Persons (AARP).
Hazell, J. (2023). Large language models can be used to effectively scale spear phishing campaigns. arXiv preprint arXiv:2305.06972.
Hong, J. (2012). The state of phishing attacks. Commun. ACM 55, 74–81.
Huigsloot, L. (2023, April 5). Multiple US state regulators allege AI trading DApp is a Ponzi scheme. Coin Telegraph.
IBM. (2023). What are large language models?
Insikt Group. (2023, January 26). Cyber Threat Analysis: Recorded Future.
Jakesch, M. et al. (2023) Human heuristics for ai-generated language are flawed. Proceedings of the National Academy of Sciences, 120(11): e2208839120, 2023.
Kalaydin, P. & Kereibayev, O. (2023, August 4). Bypassing Facial Recognition - How to Detect Deepfakes and Other Fraud. The Sumsuber.
Katte, S. (2023, February 21). BingChatGPT ‘pump and dump’ tokens emerging by the dozen: PeckShield. Coin Telegraph.
Kim, M., Song, C., Kim, H., Park, D., Kwon, Y., Namkung, E., Harris, I. G., & Carlsson, M. (2019). Scam detection assistant: Automated protection from scammers. 2019 First International Conference on Societal Automation (SA). https://doi.org/10.1109/sa47457.2019.8938036
Kreps, S., et al. (2022). All the news that’s fit to fabricate: Ai-generated text as a tool of media misinformation. Journal of Experimental Political Science, 9(1):104–117, 2022.
Lim, W.M., et al. (2023, February 23). Generative AI and the future of education: Ragnarok or reformation? A paradoxical perspective from management educators. The International Journal of Management Education, 21 (2). https://doi.org/10.1016/j.cogr.2023.06.001
Lin, T. et al. (2020, June 5). Susceptibility to Spear-Phishing Emails: Effects of Internet User 10.1145/3336141 Demographics and Email Content. ACM Trans Comput Hum Interact. 2019 Sep; 26(5): 32.
Lokanan, M. (2014). The demographic profile of victims of investment fraud. Journal of Financial Crime, 21(2), 226–242. https://doi.org/10.1108/jfc-02-2013-0004
Lokanan, M. (2022). The determinants of investment fraud: A machine learning and artificial intelligence approach. Frontiers in Big Data, 5. https://doi.org/10.3389/fdata.2022.961039
Lu, Z., Li, P., Wang, W., & Yin, M. (2022). The effects of AI-based credibility indicators on the detection and spread of misinformation under social influence. Proceedings of the ACM on Human-Computer Interaction, 6(CSCW2), 1–27. https://doi.org/10.1145/3555562
Majovsky, M., et al. (2023, May 31). Artificial Intelligence Can Generate Fraudulent but Authentic-Looking Scientific Medical Articles: Pandora’s Box Has Been Opened. J Med Internet Res. 2023; 25: e46924. 10.2196/46924
Mandiant (Google Cloud). (2023, August 17). Threat Actors are Interested in Generative AI, but Use Remains Limited. https://www.mandiant.com/resources/blog/threat-actors-generative-ai-limited
Nesbit, J. (2023, November 29). AI Investment Scams Are On The Rise - Here’s How To Protect Yourself. Nasdaq.
Ontario Securities Commission (2023, November 27). 4 signs of investment fraud.
Ontario Securities Commission. (2023). 8 common investment scams.
Ontario Securities Commission (2024), Artificial Intelligence and Retail Investing: Use Cases and Experimental Research.
Ontario Securities Commission. (2024). Get Smarter About Money: Recovery room scams.
Owen, Q. (2003, October 11). How AI can fuel financial scams online, according to industry experts. ABC News.
Pennycook, G., Bear, A., Collins, E. T., & Rand, D. G. (2020). The implied truth effect: Attaching warnings to a subset of fake news headlines increases perceived accuracy of headlines without warnings. Management Science, 66(11), 4944–4957.
Randall, S. (2023, November 8). Newcomers to Canada highly vulnerable to financial fraud, need advice. Wealth Professional.
Roozenbeek, J., van der Linden, S., Goldberg, B., Rathje, S., & Lewandowsky, S. (2022). Psychological inoculation improves resilience against misinformation on social media. Science Advances, 8(34).
Sakasegawa, J. (2023). AI phishing attacks: What you need to know to protect your users. Persona.
Saltz, E., Barari, S., Leibowicz, C. R., & Wardle, C. (2021). Misinformation interventions are common, divisive, and poorly understood. Harvard Kennedy School (HKS) Misinformation Review, 2(5).
Simon Fraser University. (2024). How to spot fake news: Identifying propaganda, satire, and false information (infographic taken from the International Federation of Library Associations and Institutions (IFLA)).
Snijders, C., Conijn, R., de Fouw, E., & van Berlo, K. (2022). Humans and algorithms detecting fake news: Effects of individual and contextual confidence on trust in algorithmic advice. International Journal of Human–Computer Interaction, 39(7), 1483–1494.
Spitale, G., et al. (2023). Ai model gpt-3 (dis) informs us better than humans. Preprint arXiv:2301.11924.
Statistics Canada. (2023, July 24). Self-reported fraud in Canada, 2019. https://www150.statcan.gc.ca/n1/pub/89-652-x/89-652-x2023001-eng.htm
TD. (n.d.). Telephone Services. TD Bank.
Texas State Securities Board. (2023, April 4). State Regulators Stop Fraudulent Artificial Intelligence Investment Scheme. Texas State Securities Board.
The EU’s Digital Services Act. European Commission. (n.d.).
The Globe and Mail. (2023, November 8). Experts warn growing use of AI will cause influx in phone scam calls.
Transunion (2023, September 12). Nearly Half (49%) of Canadians Said They Were Recently Targeted by Fraud; Around 1 in 20 Digital Transactions in Canada Suspected Fraudulent in H1 2023, Reveals TransUnion Canada Analysis.
Twitter. (n.d.) Addressing misleading information
U.S. Department of Justice. (2023, December 12). Two Men Charged for Operating $25M Cryptocurrency Ponzi Scheme.
Veerasamy, N., & Pieterse, H. (2022, March). Rising above misinformation and deepfakes. In International Conference on Cyber Warfare and Security (Vol. 17, No. 1, pp. 340-348).
Visual Capitalist (2024). How to spot fake news. https://www.visualcapitalist.com/how-to-spot-fake-news/
Wawanesa Insurance. (2023, July 6). New Scams with AI & Modern Technology. Wawanesa Insurance.
Yang, K. and Menczer, F. (2023, July 30). Anatomy of an AI-powered malicious social botnet. Observatory on Social Media, Indiana University, Bloomington.
Yaqub, W., Kakhidze, O., Brockman, M. L., Memon, N., & Patil, S. (2020). Effects of credibility indicators on social media news sharing intent. Proceedings of the 2020 CHI Conference on Human Factors in Computing Systems.