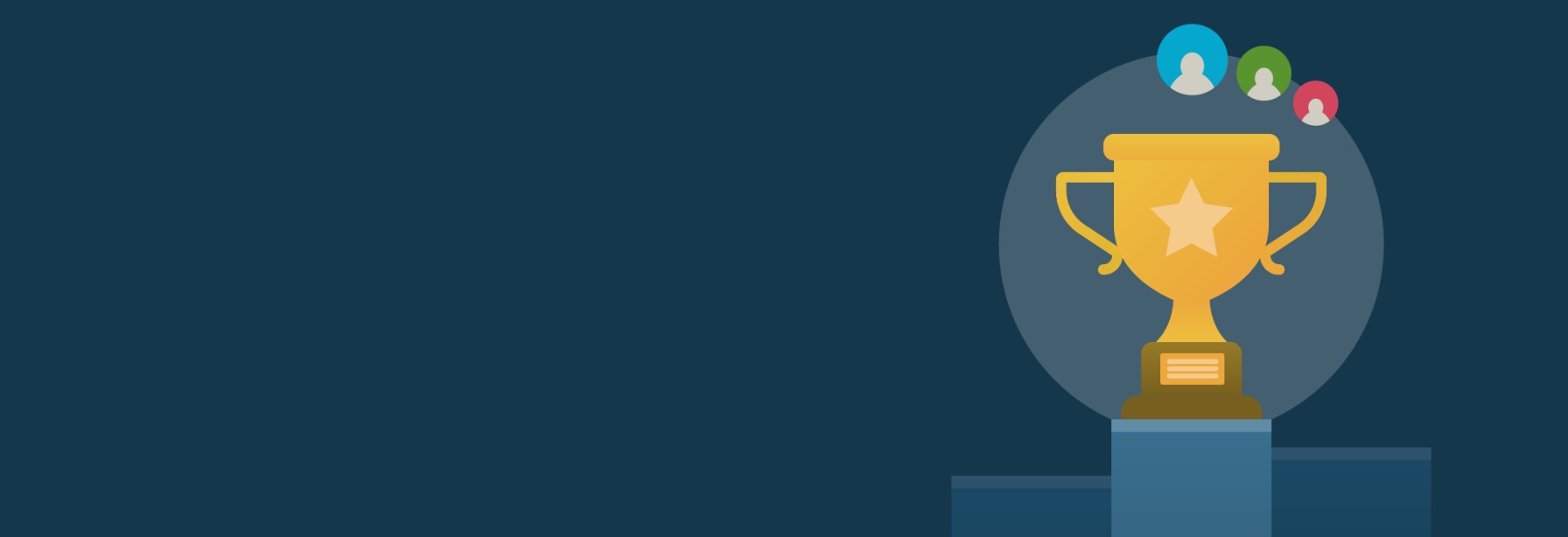
Gamification Revisited: New Experimental Findings in Retail Investing
Executive Summary
Executive Summary
As digital trading platforms have grown in popularity, regulators around the globe are alert to the usage of digital engagement practices (DEPs) within these platforms. To address this growing concern, the Ontario Securities Commission (OSC) published a 2022 research report Digital Engagement Practices in Retail Investing: Gamification and Other Behavioural Techniques.[1] The report outlines prominent gamification and other behavioural techniques (forms of DEPs) used by digital trading platforms in Canada and abroad, and also contains experimental findings focused on the effects of these techniques on retail investor behaviour.
Building on these findings, the OSC collaborated with the Behavioural Insights Team (BIT) to conduct additional research into the effects of digital engagement practices on investor behaviour. This report contains the methodology and findings from a behavioural science experiment designed to measure the impact of four tactical applications[2] of gamification and other behavioural techniques on investor behaviour:
- Social Interactions: Design elements that enable platform users to interact with other users by generating, sharing, viewing, and reacting to content, and engaging in direct messaging.
- Social Norms Data: Design features which signal social norms (i.e., information about how other users think and behave).
- Copy Trading: Platform functionality which allows users to copy the trades of other profiled users.
- Leaderboards: A public display of ranked information about users’ performance (i.e., weekly returns).
The experiment consisted of a simulated trading activity, structured in the same manner as the activity used in the OSC’s 2022 report. Participants were given $10,000 in play money and taken through ten simulated weeks of market movements in which they could buy and sell six different stocks. The experiment consisted of a control group and four treatment groups – one for each of the applications listed above.
Our primary focus was the extent to which participants traded stocks that were ‘promoted’ in the social interactions, social norms data, and copy trading conditions, as compared to the control group. This was intended to measure the influence of non-expert, social information on the trading behaviour of Canadians. Our secondary focus was on trading frequency – how many trades each experiment condition made – to assess whether the presence of digital engagement practices increases user engagement by way of elevated trading frequency.
Our experiment generated meaningful findings. With respect to trading of ‘promoted’ stocks, participants in the social interactions feed and copy trading conditions made 12% and 18% more of the total volume of their trades in the promoted stocks, respectively, compared to the control group. These differences were statistically significant. The social norms intervention did not influence this aspect of trading behaviour.
With respect to trading frequency, participants in the leaderboard group made 2.5 fewer trades than the control group (a relative difference of 14%), a statistically significant difference. This finding was surprising, and we provide additional analyses in the body of the report to explain the underlying mechanism. Participants in the social interactions feed made about 2 fewer trades than the control group (a relative difference of 11%), but this difference was not statistically significant.[3] The social norms data and copy trading conditions did not influence this aspect of trading behaviour.
The results from this experiment contribute to the OSC’s existing body of high-quality research in the area of digital engagement practices and retail investing. Based on the research findings presented in this report and the OSC’s 2022 research report, we recommend the following:
- Authorities in Canada and abroad should continue to consider whether updates to regulations and guidance for the usage of DEPs by investing platforms are required, with particular attention paid to techniques that, through high-quality research, have been demonstrated to harm investors.
- Regulators could also consider whether to limit digital trading platforms from using tactical applications of DEPs that our research indicates can compromise investor protection. Based on the OSC’s collective research, this includes points, top traded lists, social interactions feeds, and copy trading.
- Finally, we encourage authorities to gather data from firms and registrants to continue to measure the impact of DEPs on investor behaviours and outcomes. We also encourage registrants and firms that operate digital trading platforms to conduct their own research (i.e., A/B testing) to identify the effects of DEPs on the behaviour and outcomes of users. This can include both positive (i.e., diversification) and negative (i.e., high frequency trading) behaviours and outcomes, where applicable.
[1]OSC Staff Notice 11-796 Digital Engagement Practices in Retail Investing: Gamification and Other Behavioural Techniques. Ontario Securities Commission. (2022, November 17). https://www.osc.ca/sites/default/files/2022-11/sn_20221117_11-796_gamification-report.pdf
[2] By “tactical application”, we refer to how design features are presented within trading apps. These features typically leverage more than one gamification technique (e.g., “top traded lists” leverage both attention-inducing prompts and social norms). The applications tested in this experiment are based on combinations of three key gamification and other behavioural techniques: social interactions, social norms, and leaderboards.
[3] This result is trending towards but was not statistically significant (p=0.04) when using the multiple-comparison adjusted p-value of 0.0125. We used a more conservative threshold for statistical significance rather than the conventional p=0.05 to account for making four comparisons in our secondary analysis.
Introduction
Introduction
Context
In recent years there has been increased demand for digital investing platforms among retail investors in Canada and around the world. While these platforms have made it easier for retail investors to participate in financial markets, there is growing regulatory concern over the use of digital engagement practices and gamification techniques on these platforms. In response, the OSC and BIT published a 2022 research report Digital Engagement Practices in Retail Investing: Gamification and Other Behavioural Techniques. The report includes a taxonomy of nine gamification and other behavioural techniques that are currently being used by online brokerages (or may be used in the future) and their likely impact on retail investor behaviour – both positively and negatively. The report also includes results from an online experiment that examines the impact of giving investors “points” with negligible economic value for buying or selling stocks (a form of reward) and showing investors a “top traded list” (a combination of attention-inducing prompts and social norms) in a simulated trading environment. The experiment generated valuable empirical evidence with respect to the effects of these techniques on retail investor behaviours: participants who were rewarded with points made almost 40% more trades than participants who weren’t exposed to any gamification or other behavioural techniques. Furthermore, participants who were exposed to the top traded list were 14% more likely than participants in the control group to buy and sell those top listed stocks. These findings demonstrate the capacity of gamification and other behavioural techniques to significantly influence the behaviour of retail investors in ways that harm the investor experience.
Notably, in 2021 the CSA issued guidance related to the usage of gamification techniques in the advertising and marketing of crypto-trading platforms. The guidance specifically pointed to concerns around “the use of gambling-style contests, promotions or schemes such as the offering of bonuses or rewards based on the level of trading, that may encourage excessive trading by retail investors.”[4]
Research on the effects of other digital engagement practices on retail investor behaviour is valuable to help derive an evidence base that could inform investor protection efforts. The purpose of this research report is to address the evidence gap by empirically testing the effects of four more tactical applications of digital engagement practices on retail investor behaviour.
Prioritization
At the outset of our research, we conducted a prioritization exercise to select digital engagement practices to test in our experiment. We reviewed each of the nine techniques included in the taxonomy from our previous report, including gamblification, leaderboards, rewards, goal and progress framing, feedback, salience, simplification and friction, social interactions, and social norms. We then explored tactical applications of these techniques on retail investing platforms.
We prioritized tactical applications to test based on four criteria: the potential impact of the tactic on investors (based on existing evidence or hypothesized effect), the prevalence of the tactic on investing platforms, the feasibility of testing the tactic in a simulated trading environment, and the evidence gaps the research would address.
From this exercise, the following four tactical applications were selected: social interactions feed (a form of social interactions and social norms), social norms data (a form of social norms), copy trading (a form of social interactions), and a leaderboard based on investing performance. These tactical applications are based on three techniques outlined in our 2022 taxonomy – social interactions, social norms, and leaderboards – and the applications of these techniques were not tested as part of our 2022 experiment. An overview of the techniques we selected is provided below.[5]
Social Interactions
Definition: Design elements that enable platform users to interact with other users by i) generating, sharing, viewing, and reacting to content, and ii) engaging in direct messaging.
Potential risk and impact on behaviour: Greater social interactivity has been shown to lead to higher user engagement with digital applications. This may be problematic, as investors who check the status of their investments more often are shown to trade more and have worse performance.[6] Social interactivity might also contribute to problematic levels of engagement in other ways. For example, social interactions are likely to increase the disposition effect (where investors hold onto losing investments for longer periods of time) or not sharing information about losing trades to avoid embarrassment.[7] The disposition effect is more likely to occur in stock trades than (actively managed) fund trades, as traders can assign blame for poor performance to fund managers in the case of active management.[8]
Current use on digital trading platforms: Social interactions are present across a range of digital trading platforms. For example, users of a US-based platform have the option to publicly display their portfolios and share their investment decisions with other users. Additionally, another US-based platform and a platform based in Asia feature a similar mix of social interaction features: users have access to a social news feed consisting of content posted by their peers, the opportunity to contribute to conversations highlighted under “Most popular topics'' hashtags, and the chance to follow and copy other users’ investment decisions.
Social Norms
Definition: Design features which signal social norms — i.e., information about how others think and behave. This might involve informing individuals about statistics related to relevant group behaviours (e.g., “88% of your fellow users have invested in green energy this year”) or signaling “crowd approval” through features such as “This week’s 100 most popular stocks” and “Today’s trending industries.”
Potential risk and impact on behaviour: People are deeply influenced by how others think and behave. When a particular norm is not known, being informed of what others are doing can influence peoples’ behaviour. The impact of learning about a norm is higher when that norm is surprising and the group whose behaviour people are being informed of feels relevant. Given that statistics on trading behaviours have traditionally not been shared through social channels, we hypothesize that a social norms technique will appear unique to users and shift trading activity towards the ‘popular stocks’.
Current use on digital trading platforms: Social norms are commonly leveraged on digital trading platforms. For example, a US-based platform and a Canadian-based platform display a daily ‘Top stocks’ list, which sends a signal about the stocks that are receiving increased attention. Another US-based platform presents users with a list of ‘Most popular topics’, capturing the most discussed industries and investments of the day.
While we anticipate that the types of lists of commonly traded securities noted above will remain a prevalent form of social norm interventions used on digital trading platforms, more specific and personalized social norms could certainly be pushed to users (e.g., “80% of investors like you are buying ACME”). Should they be implemented, we believe that these prompts would be just as, if not more, impactful than the lists of commonly traded securities. This is primarily due to the ability of digital engagement practices to generate increasingly more relevant and personalized interventions for users.
Leaderboards
Definition: A public display of ranked information about users’ performance. Leaderboards enable and encourage social comparison and competition.
Potential risk and impact on behaviour: Leaderboards are among the most effective gamification techniques in terms of increasing user engagement.[9] While they have not been evaluated in a trading context in the academic literature, studies conducted in other contexts suggest that they can increase user engagement with digital trading platforms. An increase in engagement might mean increases in trading frequency and risk-taking, particularly with users who are motivated by social comparisons and competition. Leaderboards may also implicitly signal a social norm around striving for and celebrating high returns.
Current use on digital trading platforms: Leaderboards are a relatively less common feature of digital trading platforms. For example, a US-based platform offers the option for social investing, allowing users to compare how they are doing with their peers by featuring on a leaderboard where users are ranked based on weighted returns within a certain time frame. Users must meet certain criteria to feature on the leaderboard, including owning at least a minimum number of holdings worth at least a certain cumulative value. This is intended to dissuade members from copying deceptive results from other users (e.g., a massive return solely from one penny stock).
In the future, digital trading platforms could implement additional leaderboards for other types of investor behaviour, such as trading frequency or even social interactions like “posts” or “likes”. Displaying a leaderboard that measures activity could increase the frequency of trading, which has been documented to diminish investor returns in the long run. Leaderboards for social interactions may be deceptively risky, given the strong influence of social feedback on behaviour. On the other hand, leaderboards for completing investor education modules, where offered by digital platforms, could enhance financial literacy.
[5] See Tregebov, S., et al. (2024). Digital Engagement Practices in Retail Investing: Gamification & Other Behavioral Techniques. Bancaria, 1. and OSC Staff Notice 11-796 Digital Engagement Practices in Retail Investing: Gamification and Other Behavioural Techniques: https://www.osc.ca/sites/default/files/2022-11/sn_20221117_11-796_gamification-report.pdf for full taxonomy.
[6] Barber, B. M., & Odean, T. (2000). Trading is hazardous to your wealth: The common stock investment performance of individual investors. Journal of Finance, 55(2), 773-806.
[7] Chaudhry, S., & Kulkarni, C. (2021, June). Design Patterns of Investing Apps and Their Effects on Investing Behaviors. In Designing Interactive Systems Conference 2021 (pp. 777-788).
[8] ibid
[9] Looyestyn, J., Kernot, J., Boshoff, K., Ryan, J., Edney, S., & Maher, C. (2017). Does gamification increase engagement with online programs? A systematic review. PloS one, 12(3), e0173403.
[4] CSA-IIROC Staff Notice 21-330 Guidance for Crypto-Trading Platforms - Requirements relating to Advertising, Marketing and Social Media Use. https://www.osc.ca/en/securities-law/instruments-rules-policies/2/21-330/csa-iiroc-staff-notice-21-330-guidance-crypto-trading-platforms-requirements-relating-advertising
Experimental Research
Experimental Research
We conducted a randomized controlled trial to assess the impact of four tactical applications of digital engagement practices on investor behaviour: (1) a social feed where platform users discuss the trades they are making, (2) social norms data displaying the number of users buying and selling each stock, (3) the option to copy the trades of a “high performing” user, and (4) a leaderboard that ranks participants based on their weekly returns. Our primary interest was in whether the first three tactics (social feed, social norms data, and copy trading) influenced which stocks the participants were trading relative to a control group that was not exposed to any of these gamification or other behavioural techniques. This allowed us to measure the degree to which the trading behaviour of retail investors is influenced by non-expert, “social” content. We also examined how all four tactics influenced participants’ trading frequency, relative to the control.
The experiment was conducted online in a simulated trading environment, using Predictiv, BIT’s proprietary platform for online experimentation. Our sample included 3,509 Canadian residents from all provinces, aged 18 years and older. 59% of the sample consisted of current investors, and 54% of the sample completed the experiment on a mobile device. The sample was well-balanced on gender (54% non-male) and age (median age: 43 years).
Table 1: Experiment Overview
Condition | Description |
---|---|
Control | Participants traded six fictitious stocks over seven simulated “weeks” of trading on a platform that did not include any behavioural techniques. |
Treatment 1 (T1): Social Interactions Feed | Participants traded in the same simulated trading activity as in the Control while exposed to a “social feed” with fictitious posts from other platform traders. |
Treatment 2 (T2): Social Norms Data | Participants traded in the same simulated trading activity as in the Control while exposed to “social norms data” showing each stock and how many platform traders bought or sold that stock in the last week. |
Treatment 3 (T3): Copy Trading | Participants traded in the same simulated trading activity as in the Control while exposed to the buy and sell activity of a “high performing” trader on the platform. |
Treatment 4 (T4): Leaderboard | Participants traded in the same simulated trading activity as in the Control while exposed to a leaderboard, which displayed their weekly return (%) compared to other platform traders. |
Research participants received $10,000 in simulated “money” to invest in up to six different fictitiously named stocks on a made-up trading platform named Stockland. After their initial allocation of funds, they were taken through seven simulated weeks of price movements, with an option to buy and/or sell stocks between each week. At the end of the experiment, all participants received a fixed amount of approximately $2.00 for participating in the experiment. They also earned up to $1.10 in additional compensation based on their final portfolio value at the end of the experiment. Participants were aware that the larger their portfolio value at the end of the experiment, the more they would earn. This created an incentive for participants to trade thoughtfully and to try to maximize their returns.
Price movements for the fictitious stocks used in our simulation were based on real stocks. We randomly selected stocks that met the following criteria: (1) listed on the Nasdaq exchange website, (2) within the technology sector, (3) with a micro ($50M-$300M) or nano (<$50M) market capitalization, and (4) an opening price below $4 per share. For the selected stocks, we used historical closing price data for eight consecutive Mondays in 2021 to determine the opening prices for each of the weeks of the experiment. The objective was to offer participants the ability to trade stocks with a similar risk profile and a medium-high level of volatility. Participants were informed at the onset of the simulation that all stock price movements were based on real, historical data.
The primary objective of the experiment was to assess whether investing platforms promoting certain assets present a risk to investors. Therefore, we promoted particular stocks each week and assessed how closely participants were following these promotions – based on their trading behaviour. The same stocks were “promoted” across the social interactions condition, social norms condition, and copy trading condition in each week. These stocks were promoted as either a “buy” or “sell” and, if followed correctly, resulted in the participant’s portfolio gaining value from week 0 to week 2, then alternating between gains and losses from weeks 3 to 7. We also varied how strongly the buy and sell indications were signalled – ranging from “strong sell” to “strong buy”.
The stocks were promoted in the following ways in each condition:
- Social Interactions Feed (T1): Promoted stocks were featured within the posts on the feed (e.g., “Ciber taking a hit on the market today – going to get out while I can” to promote a sell, or “Boodl has been killing it these last few weeks – potential for BIG gains” to promote a buy).
- Social Norms Data (T2): All stocks available for trading were displayed in a ‘Market Movers’ table outlining the number of buys and sells for each stock. Promoted stocks had a higher number of users who bought or sold these stocks: promoted stocks had at least 1000 people buying or selling the stock, while non-promoted stocks had, at most, 600 people buying or selling the stock.
- Copy Trading (T3): Promoted stocks were featured in the trader’s weekly trading activity, including whether they were a buy or sell and the dollar amount of each trade. Non-promoted stocks were displayed as a hold.
Social Interactions Feed
This arm was modelled on the interface of a platform based in Asia, where participants can engage in online discussions with other users. During the trading activity in our experiment, participants were exposed to a “social feed” with fictitious posts from other Stockland users (Figure 1). The social feed was updated weekly and included four posts by other Stockland users. The majority of the posts referenced one of the promoted stocks, displaying the users’ sentiment and/or trading behaviour with respect to the stock. One post each week featured more general investment content (i.e., not specific to any one stock), to increase the realism of the feed. The posts were designed to mimic the format of common social media websites (e.g., Twitter/X), and included the user’s name (first name and initial), a profile picture, and the number of likes and comments the post received.
We hypothesized that participants would be more likely than the control group to buy and sell the stocks referenced in the social feed posts. We also hypothesized that participants would increase their overall trading frequency as a result of being exposed to the feed.
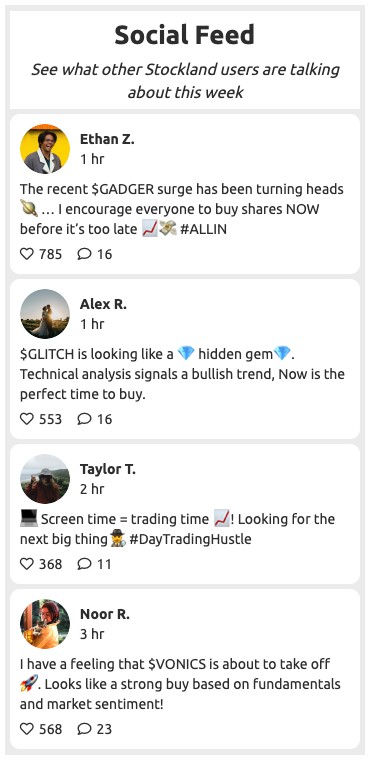
Figure 1: Adapted Social Interactions Feed
Social Norms Data
This condition was modelled on the interface of a Canadian-based platform. In this condition of our experiment, participants were exposed to a “Market Movers” dashboard throughout the trading activity (Figure 2). The dashboard displayed the number of Stockland users that bought and sold each stock each week.
We hypothesized that participants would be influenced by the group activity signalled by the dashboard and would be more likely to buy and sell the stocks that had the highest amount of buy and sell activity from other Stockland users. As a result of following the trading activity of other uses, we also hypothesized that participants would increase their trading frequency.
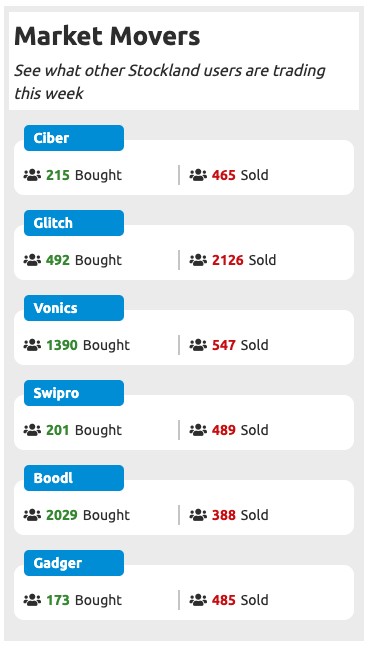
Figure 2: Adapted Social Norms Data
Copy Trading
This arm was modelled on the copy trading feature of a platform based in Asia, where participants can choose between a number of different profiled traders and copy their trades. In this condition of our experiment, participants were able to view and copy the trades of a profiled “high performing” Stockland user (Figure 3). Participants were first introduced to the ‘copy trader’ in week 1 and shown a short explanation of the trader’s investing profile. They were then shown the exact trades (i.e., dollar amount) that the trader was making each week, along with some brief commentary from the trader.
We hypothesized that participants would be influenced by the behaviour of the copy trader and be more likely to follow the trades that the copy trader made. As a result of copying the trades of a relatively high-frequency trader, we also hypothesized that participants would, by extension, increase their trading frequency.
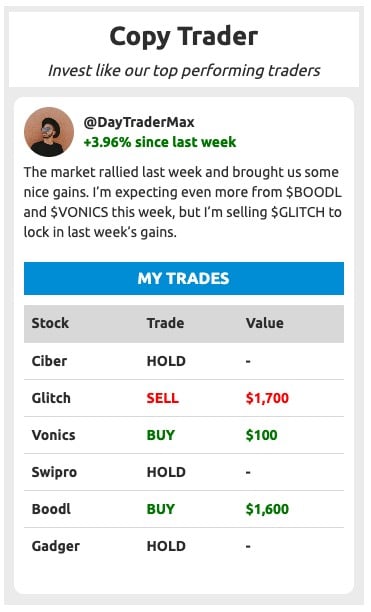
Figure 3: Adapted Copy Trading
Leaderboard
This condition was modelled on the leaderboards displayed on a US-based platform. In this condition of our experiment, participants were exposed to a leaderboard that ranked Stockland users based on their weekly returns (Figure 4). Data from our 2022 experiment was used to rank participants’ performance based on their weekly return (%). To enhance realism, the other users on the leaderboard were given names (first name and last initial) and a profile picture. Participants were introduced to the leaderboard in week 0 and appeared on the leaderboard in each subsequent week of the activity.
We hypothesized that participants would try to actively improve their position on the leaderboard by increasing their weekly returns. In doing so, we hypothesized that participants would increase their trading frequency.
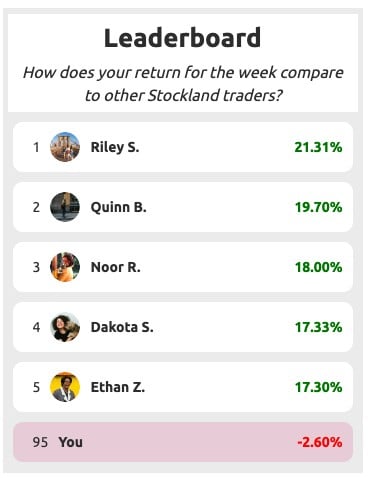
Figure 4: Adapted Leaderboard
After finishing all seven simulated weeks of trading, participants were shown their final portfolio value and asked a few questions about their demographics. They were then asked for any additional feedback on the study and given a link to claim their variable reward.
Our experiment was designed to be as applicable as possible to real world trading. Beyond the use of variable compensation and modelling asset price movements using real data, our experiment methodology (randomized controlled trial) enabled us to measure the individual effects of social interactions, social norms, copy trading, and leaderboards on trading behaviour. Overall, the trial implementation was successful:
- Recruitment was effective and the sample size was sufficiently large, with approximately 700 participants in each condition.
- Participants engaged meaningfully with the trading simulation, with a median completion time above 12 minutes.[10]
[10] Data from the handful of participants who completed the experiment in less than 1/5th of the average time was excluded from the analysis, as pre-specified in our trial protocol.
This section describes the findings of our experiment testing how four tactical applications of digital engagement practices influence trading behaviour. Our findings can be summarized as follows:
Primary Results: Trading Promoted Stocks
- Participants in the social interactions feed and copy trading conditions made 12% and 18% more of the total volume of their trades in the promoted stocks, respectively, compared to the control group. These differences were statistically significant.
- The social norms intervention did not influence this aspect of trading behaviour.
Secondary Results: Trading Frequency
- Participants in the leaderboard group made about 2.5 fewer trades than the control group (a relative difference of 14%), a statistically significant difference.
- Participants in the social interactions feed made about 2 fewer trades than the control group (a relative difference of 11%).[11]
- The social norms data and copy trading conditions did not influence this aspect of trading behaviour.
Primary Results: Trading Promoted Stocks
Our primary outcome of interest was the percentage of trades in the stocks being promoted on any given round of trading through the social interactions feed, social norms data, or copy trader. We focused on this outcome to understand the extent to which retail investors are meaningfully influenced by non-expert, “social” content. In all relevant conditions (social interactions, social norms, and copy trading), we hypothesized that participants would trade more heavily in the promoted stocks.
As shown in Figure 5 below, participants in the social interactions feed and copy trading conditions made 12% and 18% more of the total volume of their trades in the promoted stocks, respectively, compared to the control group. These differences were statistically significant. The social norms intervention did not influence this aspect of trading behaviour.
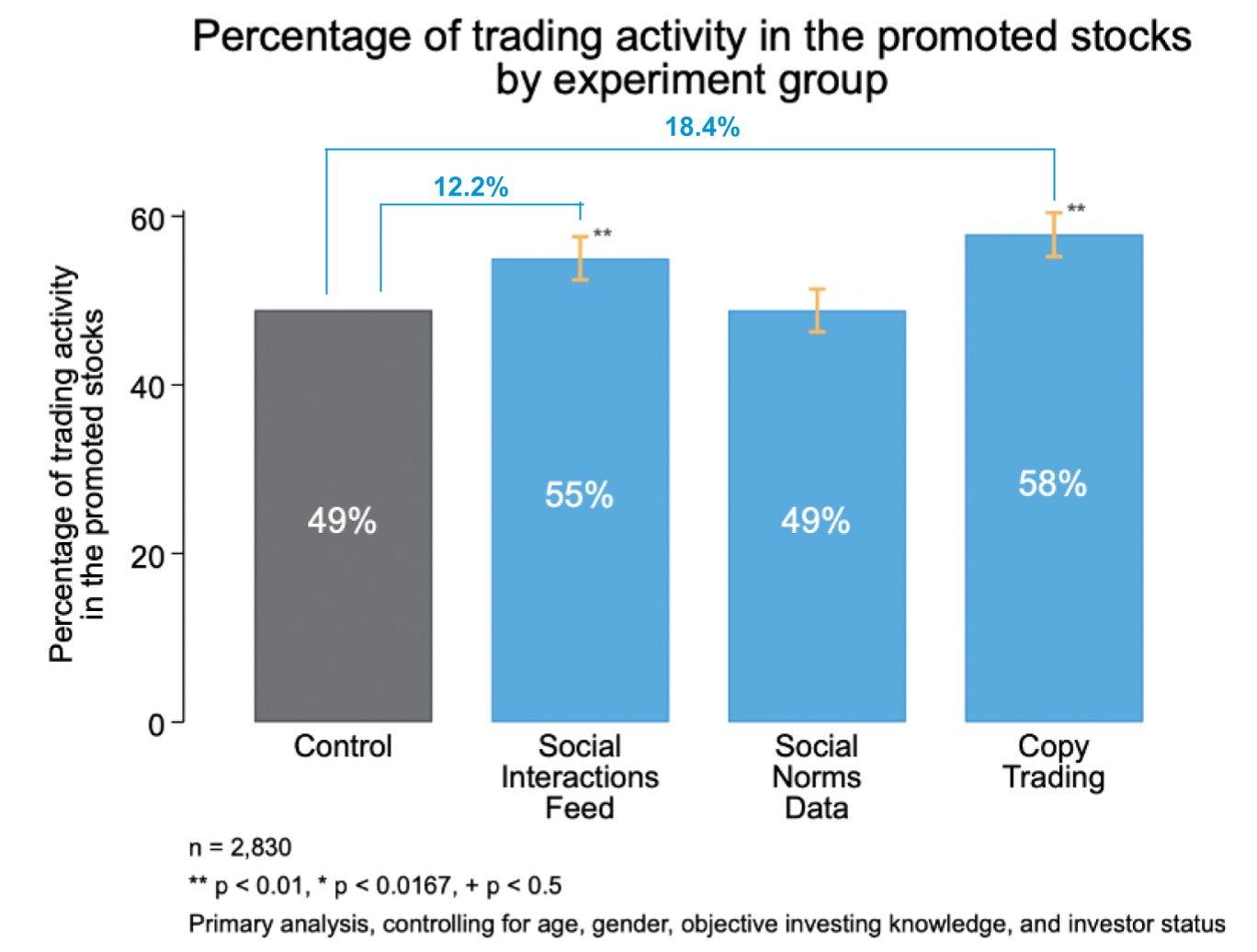
Figure 5: Trading of Promoted Stocks by Experiment Group
These findings suggest that socially based engagement techniques can meaningfully influence investor behaviour by encouraging trading in specific assets. This influence is likely to have a negative impact, potentially through under-diversification or excessive risk taking. Research has shown that when retail investors concentrate their activity on popular stocks – called “herding” – their returns are lower.[12] Other research studies further suggest that social interactions and copy trading increase risk taking; Several experiments in recent years have illustrated this effect for copy trading. [13][14][15] In addition, an analysis of a large sample of trading records from a brokerage that allows investors to interact with their peers in a social media like setting (i.e., social feed) found that investor risk-taking increases as a result of their peers’ (positive) trading performance.[16]
We believe that the amount of mental effort required to engage with the different techniques in our experiment explains much of the difference in their influence on trading behaviour. It is likely that copy trading had the largest impact on participants’ trading decisions because it provided the clearest and most direct information; the exact amounts bought and sold each week. Participants needed to expend very little effort to take up the suggestions. Following this logic, the social interactions feed had a slightly lower impact because the guidance it provided was slightly less direct and slightly more complex. Finally, the social norms data, which required the most cognitive effort to interpret and translate into investing direction, had no effect at all.
Secondary Results: Trading Frequency
Our secondary outcome of interest was trading frequency. Given the potential incentive for digital trading platforms to encourage more trading and the negative impact of higher trading frequency on investor returns,[17] we wanted to test whether exposure to the selected digital engagement techniques increase the trading frequency of participants. We measured trading frequency as the number of times participants either bought or sold a stock over the course of the trading simulation, not including the initial allocation in week 0.
As shown in Figure 6, participants in the leaderboard group made about 2.5 fewer trades than the control group (a relative difference of 14%), which was statistically significant. Participants in the social interactions feed made about 2 fewer trades than the control group (a relative difference of 11%).[18] Participants in the social norms data and copy trading conditions did not significantly alter their trading frequency.
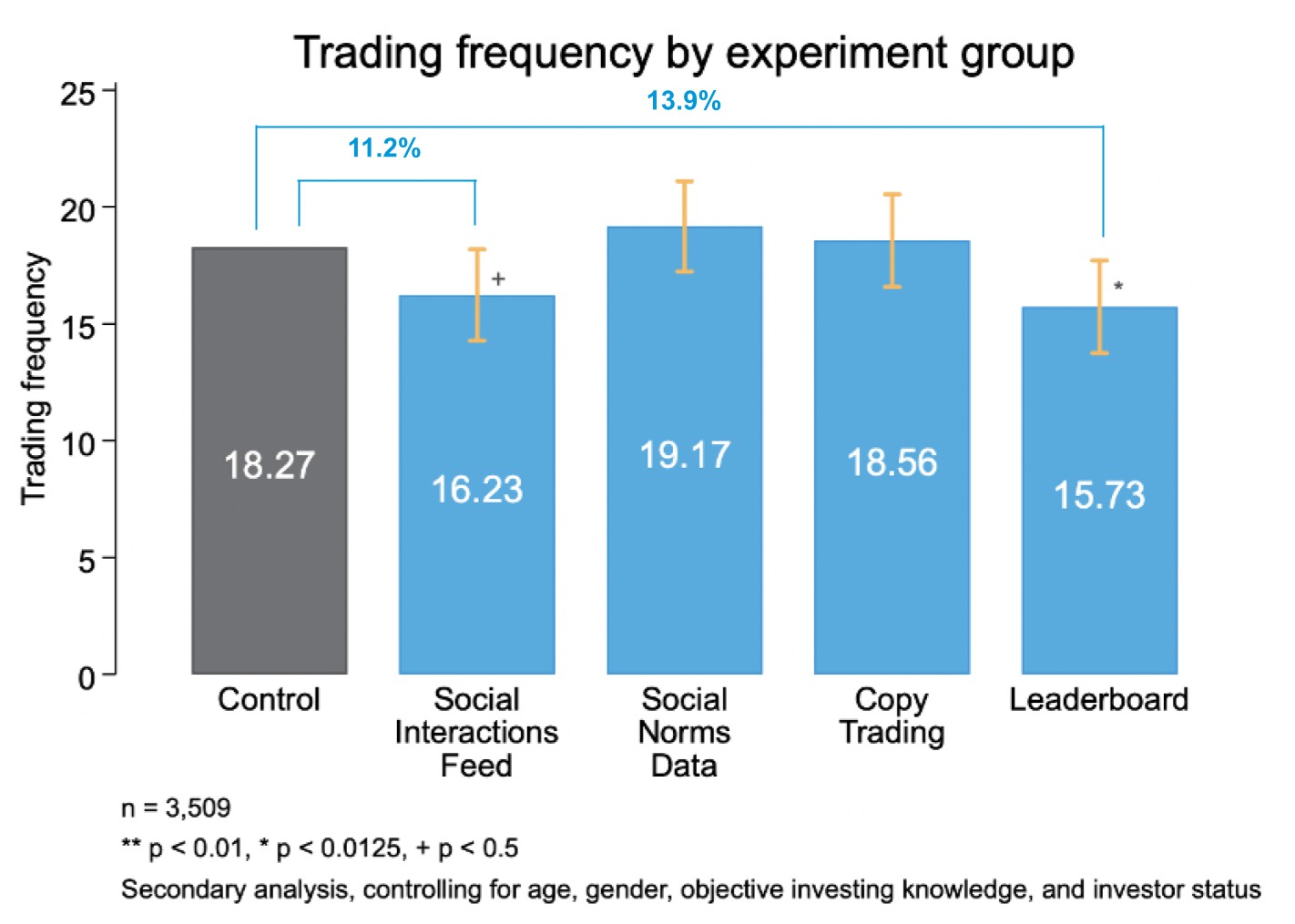
Figure 6: Trading Frequency by Experiment Group
We hypothesized that the leaderboard would increase participants’ trading frequency. We anticipated that the leaderboard would motivate risk-taking behaviour of participants as they attempted to increase their weekly returns. We believed that this effect would be operationalized in trading frequency. A recent lab experiment found that having a leaderboard in a simulated investing activity increases risk-taking.[19] We analyzed the data to explore potential explanations for this surprising effect. First, we found that investors in the leaderboard condition did not make larger trades (i.e., higher dollar value) – a mechanism by which participants could have taken on more risk. A second explanation may be that leaderboards encourage investments in higher risk securities – which is not something that our experiment allowed us to measure as all of the stocks were fundamentally similar. Finally, it is possible that the trading behaviour of participants depended on where they sat on the leaderboard. An exploratory analysis of the impact of leaderboard position on trading volume yielded the results in Figure 7. As illustrated, those in the highest and lowest positions on the leaderboard traded less, while those in the middle positions traded more.[20] This suggests that the impact of leaderboards on trading frequency is mediated by leaderboard position, with an overall reduction in trading frequency masking a more nuanced relationship. Further research may be warranted to confirm this explanation.
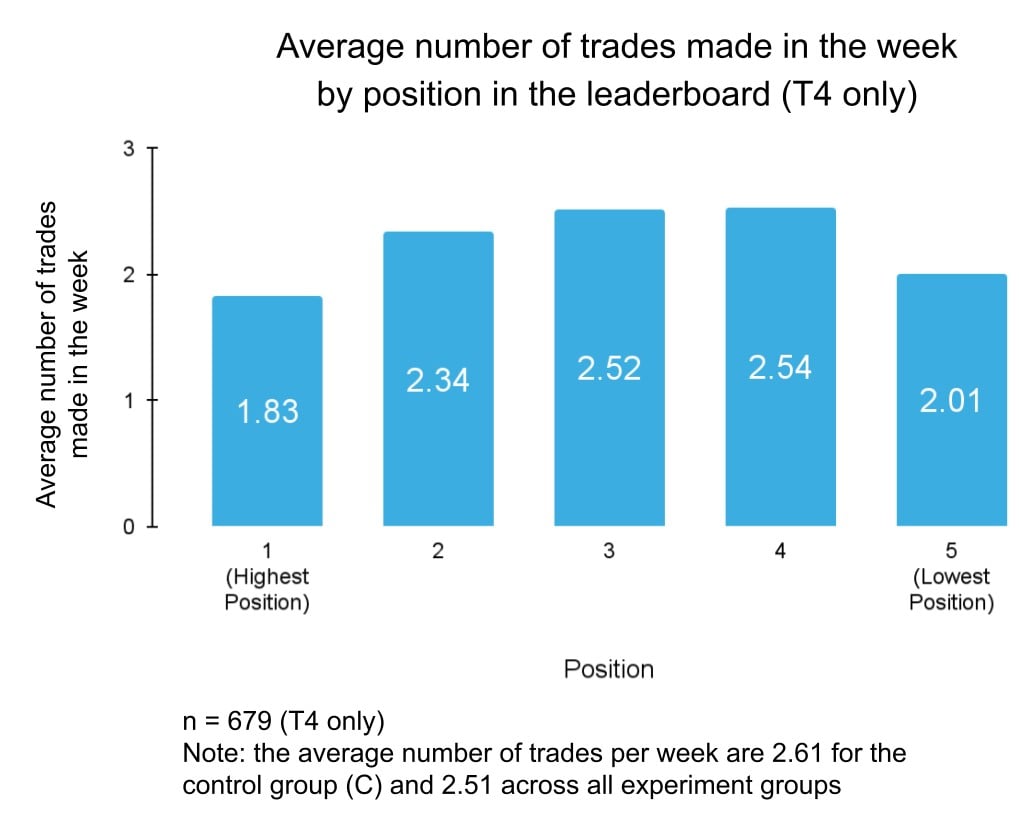
Figure 7: Number of Trades by Leaderboard Position
We had also hypothesized that participants in the social interactions feed and copy trading conditions would increase their trading frequency, relative to the control. It appears, however, that participants substituted trading behaviour into the promoted stocks, rather than increasing it to trade the promoted stocks. We were particularly surprised by the null result for copy trading, as the highlighted trader made 22 trades after the initial allocation in week 0, which was higher than the control group average of 18. Again, we note that participants in our experiment were required to manually copy the trades (i.e., trading was not automated), which may have moderated their activity.
Limitations
When interpreting the results from this experiment, a few limitations should be noted. First, our copy trading arm arguably made it more challenging for participants to copy trade than in the real world. Participants had to manually enter trades, and could only copy one trader. In reality, investors can automatically copy trades from many different traders. Given this, we believe that our results understate the effects of copy trading in the real world. Second, we presented social norms data to participants in quite a neutral fashion, and did not include any design features (i.e., colour, stock ordering) that may guide behaviour. Therefore, we do not believe that these results indicate that social norms do not influence the trading behaviour of investors, but that the specific implementation of social norms we selected is less likely to influence trading behaviour. Finally, participants in our experiment had limited access to information about each stock. In a real world setting, they would have access to a range of fundamental and technical indicators. We believe the limited availability of other data may have made the social feed and copy trading information somewhat more salient and influential. Despite these limitations, we are confident that the design and execution of our experiment generated results that are indicative of real world investor behaviour.
[11] This result is trending towards but was not statistically significant (p=0.04) when using the multiple-comparison adjusted p-value of 0.0125. We used a more conservative threshold for statistical significance rather than the conventional p=0.05 to account for making four comparisons in our secondary analysis.
[12] Barber, B. M., Huang, X., Odean, T., & Schwarz, C. (2022). Attention‐induced trading and returns: Evidence from Robinhood users. Journal of Finance, 77(6), 3141-3190.
[13] Broihanne, M. H. (2023). GAMIFICATION AND COPY TRADING IN FINANCE: AN EXPERIMENT1.
[14] Andraszewicz, S., Kaszás, D., Zeisberger, S., & Hölscher, C. (2023). The influence of upward social comparison on retail trading behaviour. Scientific Reports, 13(1), 22713.
[15] Apesteguia, J., Oechssler, J., & Weidenholzer, S. (2020). Copy trading. Management Science, 66(12), 5608-5622.
[16] Klocke, N., Müller, D., Hasso, T., & Pelster, M. (2022). The impact of peer performance on individual investors’ risk-taking. HKIMR Working Paper 19.
[17] Barber, B. M., & Odean, T. (2000). Trading is hazardous to your wealth: The common stock investment performance of individual investors. Journal of Finance, 55(2), 773-806.
[18] This result is trending towards but was not statistically significant (p=0.04) when using the multiple-comparison adjusted p-value of 0.0125. We used a more conservative threshold for statistical significance rather than the convention p=0.05 to account for making four comparisons in our secondary analysis.
[19] Hüller, C., Reimann, M., & Warren, C. (2023). When Financial Platforms Become Gamified, Consumers’ Risk Preferences Change. Journal of the Association for Consumer Research, 8(4), 429-440.
[20] Note that the “position” refers to quintiles. Position 1 represented the top 20% of performance and received a leaderboard ranking of 10-16; Position 2 represented the next 20% and received a ranking of 32-38; Position 3 had a ranking of 52-58; Position 4 a ranking of 72-78; and Position 5 a ranking of 92-98.
Conclusion & Recommendations
Conclusion & Recommendations
In 2022, the Ontario Securities Commission (OSC) published the report Digital Engagement Practices: Gamification & Other Behavioural Techniques. This report set out a taxonomy of nine techniques currently employed on investing platforms or with high relevance to retail investing. The 2022 report also included the results of an online randomized controlled trial showing the impact of “points” and “top-traded lists” on trading volume and the trading of specific stocks.
This report builds on the 2022 report with a follow-up experiment that tested the impact of four additional digital engagement practices related to social norms, social comparisons (e.g., leaderboards), and social interactions. These practices were selected for testing given the proliferation of social features on investing platforms, the potential risk of harm they pose to investors, and the lack of high-quality evidence on their impact.
Our experimental methodology mirrored that which was employed in the 2022 report. Participants took part in a simulated activity that allowed them to trade a selection of stocks. They were randomly assigned to either a control group or one of four treatment groups featuring a digital engagement technique: copy trading, a social interactions feed, social norms data, or a leaderboard. We analyzed the impact of these techniques on the trading behaviour of participants to identify any investor harm associated with the usage of these techniques on digital investing platforms.
We found that copy trading and a social interactions feed had a significant impact on trading behaviour. Participants shifted their investing decisions to mimic the profiled trader in the copy trading arm and to buy or sell the stocks promoted in the social feed. The effects were particularly large in the copy trading group. This represents a significant risk to the retail investor experience, as “herding” (when a large number of retail investors collectively move into or out of a stock at the same time) has been shown to negatively impact investor returns.
Based on the research findings presented in this report and the OSC’s 2022 research report, the OSC’s Research and Behavioural Insights Team recommend the following:
- Authorities in Canada and abroad should continue to consider whether updates to regulations and guidance for the usage of DEPs by investing platforms are required, with particular attention paid to techniques that, through high-quality research, have been demonstrated to harm investors.
- Regulators could also consider whether to limit digital trading platforms from using tactical applications of DEPs that our research indicates can compromise investor protection. Based on the OSC’s collective research, this includes points, top traded lists, social interactions feeds, and copy trading.
- Finally, we encourage authorities to gather data from firms and registrants to continue to measure the impact of DEPs on investor behaviours and outcomes. We also encourage registrants and firms that operate digital trading platforms to conduct their own research (i.e., A/B testing) to identify the effects of DEPs on the behaviour and outcomes of users. This can include both positive (i.e., diversification) and negative (i.e., high frequency trading) behaviours and outcomes, where applicable.
More broadly, we believe that our 2022 report and this follow-up research demonstrate the significant value of high-quality empirical evidence for assessing risk and establishing evidence-informed approaches to securities regulation. We encourage stakeholders to continue to invest in, publish, and disseminate online trials, field experiments, and analyses of historical trading data that shed light on the impact of emerging digital engagement practices on the investor experience.
Authors & Appendices
Authors
Ontario Securities Commission:
Patrick Di Fonzo
Senior Advisor, Behavioural Insights
[email protected]
Matthew Kan
Senior Advisor, Behavioural Insights
[email protected]
Meera Paleja
Program Head, Research and Behavioural Insights
[email protected]
Kevin Fine
Senior Vice President, Thought Leadership
[email protected]
Behavioural Insights Team (BIT):
Laura Callender
Senior Advisor
[email protected]
Riona Carriaga
Associate Advisor
[email protected]
Sasha Tregebov
Director
[email protected]
Figure 1: Initial Trading Screen
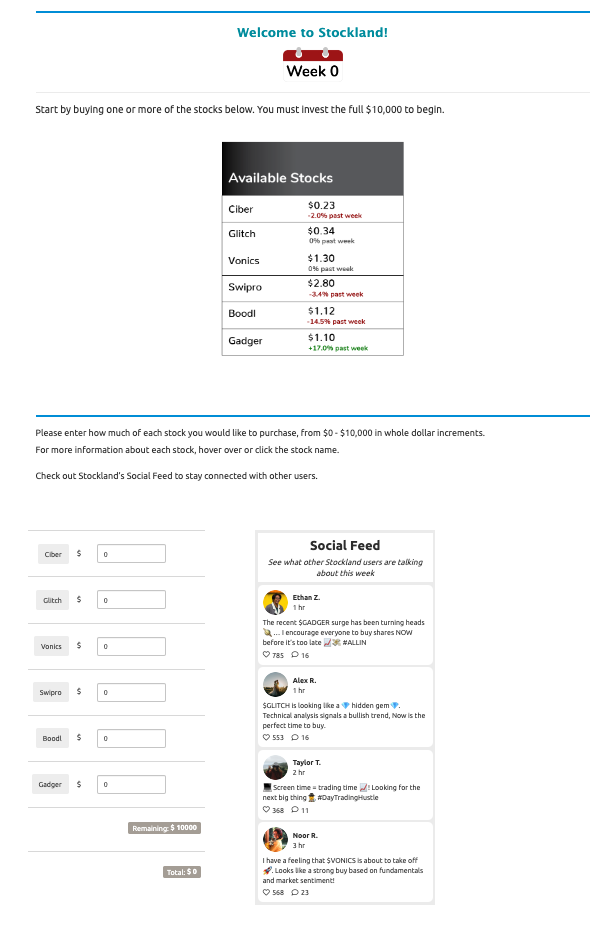
NOTE: This was the display for participants in the social interactions feed condition. Those in the social norms, copy trading, and leaderboard conditions would have seen information relevant to that condition. Participants in the control group only saw the trading screen.
Figure 2: Sell Screen
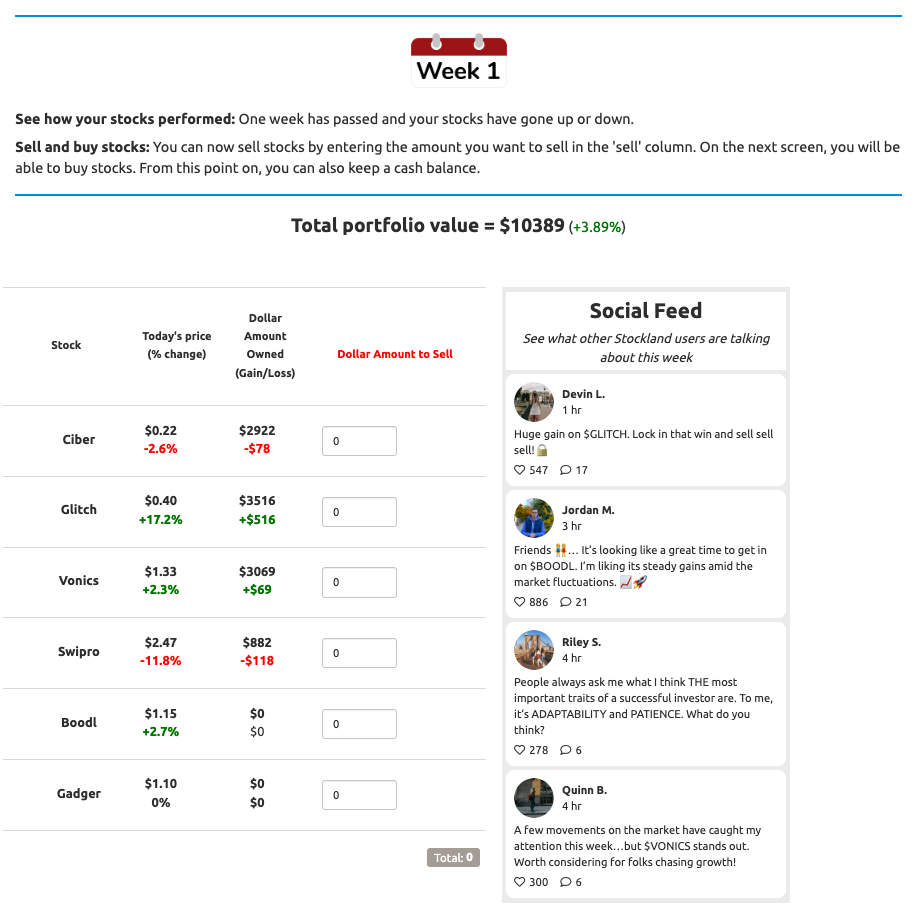
NOTE: This was the display for participants in the social interactions feed condition. Those in the social norms, copy trading, and leaderboard conditions would have seen information relevant to that condition. Participants in the control group only saw the trading screen.
Figure 3: Buy Screen
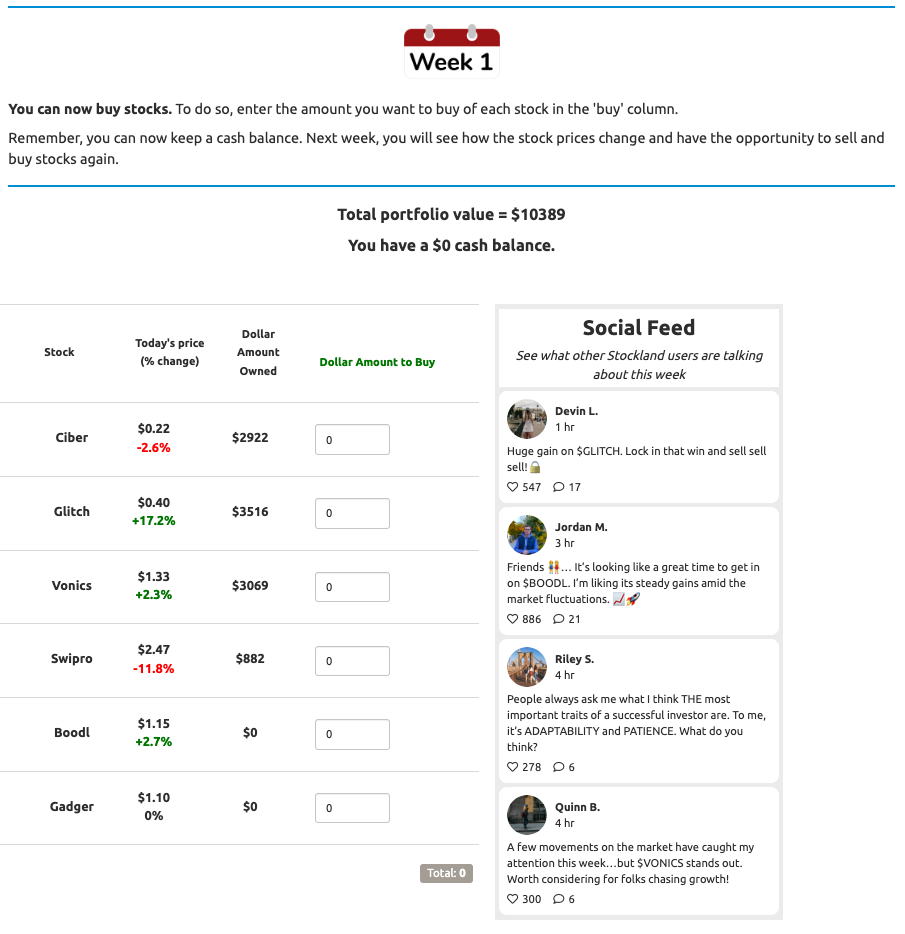
NOTE: This was the display for participants in the social interactions feed condition. Those in the social norms, copy trading, and leaderboard conditions would have seen information relevant to that condition. Participants in the control group only saw the trading screen.
Andraszewicz, S., Kaszás, D., Zeisberger, S., & Hölscher, C. (2023). The influence of upward social comparison on retail trading behaviour. Scientific Reports, 13(1), 22713.
Apesteguia, J., Oechssler, J., & Weidenholzer, S. (2020). Copy trading. Management Science, 66(12), 5608-5622.
Barber, B. M., Huang, X., Odean, T., & Schwarz, C. (2022). Attention‐induced trading and returns: Evidence from Robinhood users. Journal of Finance, 77(6), 3141-3190.
Barber, B. M., & Odean, T. (2000). Trading is hazardous to your wealth: The common stock investment performance of individual investors. Journal of Finance, 55(2), 773-806.
Broihanne, M. H. (2023). GAMIFICATION AND COPY TRADING IN FINANCE: AN EXPERIMENT1.
Chaudhry, S., & Kulkarni, C. (2021, June). Design Patterns of Investing Apps and Their Effects on Investing Behaviors. In Designing Interactive Systems Conference 2021 (pp. 777-788).
CSA-IIROC Staff Notice 21-330 Guidance for Crypto-Trading Platforms - Requirements relating to Advertising, Marketing and Social Media Use. https://www.osc.ca/en/securities-law/instruments-rules-policies/2/21-330/csa-iiroc-staff-notice-21-330-guidance-crypto-trading-platforms-requirements-relating-advertising
Hüller, C., Reimann, M., & Warren, C. (2023). When Financial Platforms Become Gamified, Consumers’ Risk Preferences Change. Journal of the Association for Consumer Research, 8(4), 429-440.
Klocke, N., Müller, D., Hasso, T., & Pelster, M. (2022). The impact of peer performance on individual investors’ risk-taking. HKIMR Working Paper 19.
Looyestyn, J., Kernot, J., Boshoff, K., Ryan, J., Edney, S., & Maher, C. (2017). Does gamification increase engagement with online programs? A systematic review. PloS one, 12(3), e0173403.
OSC Staff Notice 11-796 Digital Engagement Practices in Retail Investing: Gamification and Other Behavioural Techniques. Ontario Securities Commission. (2022, November 17). https://www.osc.ca/sites/default/files/2022-11/sn_20221117_11-796_gamification-report.pdf
Tregebov, S., et al. (2024). Digital Engagement Practices in Retail Investing: Gamification & Other Behavioral Techniques. Bancaria, 1. and OSC Staff Notice 11-796 Digital Engagement Practices in Retail Investing: Gamification and Other Behavioural Techniques: https://www.osc.ca/sites/default/files/2022-11/sn_20221117_11-796_gamification-report.pdf